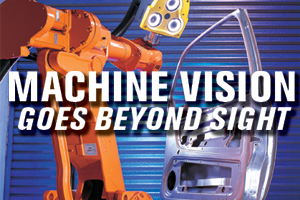

Gaging the location of a car hood in a robotic workcell was the task of the machine-vision system. A car manufacturer had a requirement in which car hoods would be brought in for final assembly and 50 to 100 of the hoods would be stacked on rails. But the task wasn’t easy.
Forklifts were used to load the hoods onto the rails, but they were not put in a reliable position for the robots to pick up. Each hood was at a different angle, each part slightly askew. Its precise location had to be found because of the tight tolerance required when loaded onto a metal fixture that readied the part for further processing.
“The tolerance was so tight that when a human operator took the hood off the rails and put it in the carriage fixture so that the set pin would go through the hole on the car roof, it would make a loud screeching noise,” says Phil Heil, application engineer for DVT Sensors (Duluth, GA). “With every cycle, you would swear that the robot had crashed.”
To solve the problem, the company integrated a machine-vision system that used special lighting and multiple cameras that were linked together. Strobe lighting backlit the parts, shining in off the sides, and the cameras were able to capture the angle of the hood as well as an X and Y position. The cameras, two of which were linked to a master camera and which “spoke” to each other via the Ethernet, were able to get the position of the car hood down to 0.002 of an inch.

One example
This machine-vision application is just one example of what can be accomplished with this technology, but it in no way utilizes the technology to its fullest. Today’s machine-vision systems feature capabilities that go way beyond this application. In fact, this system that is already in place is capable of expanding to include many other tasks.
“It would be pretty straight forward to move the hood around and take several inspections,” says Heil. “That way, it is almost like a CMM (coordinate measuring machine) at that point.”
This range of capabilities is typical, many machine-vision experts say. Zvika Rotem, vice president of marketing for CogniTens Ltd., (Ramat HaSharon, Israel), says, “Today’s systems are ‘intelligent.’ They detect and classify defects and problems, and support automated go/no-go decisions. These capabilities are applied both for 2-D and 3-D vision systems, and play an important role in any industrial application that needs tight process control and immediate feed back for process corrections.”
An additional trend is the improvement in image acquisition time, making systems insensitive to environmental disturbances. That is one reason the cameras at the car factory were linked, Heil says. Linking them negates the effects of vibration and improves throughput.
Another aspect of today’s machine-vision systems is the advancement in “self learning,” says Ben Dawson, director of strategic development at Coreco Imaging (Billerica, MA). “Machine learning has been around for a long time,” he says. “But, the technology of machine learning has matured to the point where now we can apply it. It is not a research project that we would put a graduate student to work on. Today’s systems have very good tools and computers that have increased power to run them.”
Measurement capabilities
The dimensional measurement capability that manufacturers can expect might surprise some end users, the experts say. Measurement capabilities for industrial manufacturing applications include measurement of features such as holes and edge points, measurement of
single-surface points on parts, full-
surface measurements, measurement of distances between features and angles, and full and accurate high-quality clouds of points.
“The tools in the camera allow you to locate edges and, based on those edges, generate measurements,” says Heil. “After those measurements are computed, what we’ll normally do is put down either a golden standard that has been measured with another device or use a traceable standard and put it in the field of view. We can then get a scaling from millimeters or inches into pixels and put that into the system.”
Software is key, and processing power is making many of these capabilities possible. “All these measurements must be performed at a very high accuracy,” says Rotem. “The obtained results are compared to the original mathematical model of the measured part, and the machine-vision system must offer a variety of different analytical tools.”
Ken MacDonald, applications engineer for Pulnix America Inc. (Sunnyvale, CA), adds that cameras offer great performance characteristics that are useful for metrology applications. “One performance characteristic is pixel-to-pixel stability, which is the ability to address each pixel without pixel jitter. That adds the ability to perform a subpixel measurement,” he says. “Signal-to-noise characteristics are also improved and that gives the end user the ability to differentiate between more signal information and less noise. Also the increase in pixel resolution at a reasonable cost is a reality today.”
Some companies offer progressive scan cameras and that, MacDonald says, means the user may no longer need to use strobe lights to achieve full-frame resolution. “Progressive scan technology adds the ability to asynchronously shutter capture a fast moving object at a frame resolution that in the past required a strobe light,” he says. He adds that partial scanning can increase frame rates.
For example, if using a 640- by 480-pixel resolution camera that runs at 60 frames per second and the user wants to increase the frame rate, they can do so by using a partial scan mode of 640 pixels by 100 lines and that will increase the frame rate to 222 frames per second. “The only issue here is that the field of view is reduced to 100 lines,” MacDonald says, “but if you are inspecting a widget you really do not require the full vertical resolution as much as you would require the horizontal resolution due to the parts characteristic.”
Another machine-vision capability is looking at non-metric kinds of defects. While most measurements focus on holes and distances, in some cases the defects can’t be specified. Detecting scratches, cracks or other surface flaws would be a non-metric defect. “In this case, the user would want to say something like ‘must not be more than X in length,’ but say nothing about where it is, the depth or the shape of the flaw,” says Dawson. “We can’t specify all the possible defects by a set of metrics, but the new machine-vision systems are able to deal with these kinds of non-metric inspections.”
Not always the end all
Machine-vision systems range in their dimensional measurement capabilities especially when comparing those used in offline applications to those used in in-process applications, says Dawson. “Off-line systems are often combined with sophisticated optics to give very high precision, accuracy and repeatability, but at a substantial price,” he says. “On-line or in-process systems typically have to settle for less precision, accuracy and repeatability as they can’t carefully control the part placement, take a long time to measure it and they have to be inexpensive.”
Generally machine-vision systems work in “world pixels,” which is the size of a sensor element in a camera after it has been projected through the lens onto the part. This then becomes a “dimensionless” measure for specifying capabilities. While some manufacturers say that their systems can reach 1/40th of a pixel, Dawson disagrees, saying that it is unlikely that most end users will reach that level without a lot of careful work. “On most applications we see anywhere from 1/6th to 1/20th of a pixel in repeatability, perhaps a little less in accuracy due to lens distortion,” he says. “The precision of a machine-vision system appears to be very high about 5 or 6 digits, but this is meaningless if your repeatability and accuracy are only 1 part in 20.”
Despite, or maybe because of all the increased capabilities, some end users do have an inflated view of what machine vision systems can do. The rule of thumb is, “Can a human do this?” If a worker can’t see what the camera would see, or if the worker can’t verbalize what needs to be inspected, a machine-vision system probably won’t be able to help.
To help users understand integrating machine systems in their factory, there are a few “rules of thumb.” These have been culled from interviews with Rotem, Dawson, Heil and MacDonald.
- Is there someone who has some experience and comfort with machine vision? If not, is someone willing to learn? Even though the machine vision products are easy to use, they have to be supported by lighting, optics and mechanics (staging), and they have to connect into the existing factory automation system. Also, someone needs to be a “champion” for the use of machine vision if it is an unfamiliar technology.
- The system must be able to measure the required parts and surfaces. Is visual inspection appropriate? Do you have a clear view of the item being inspected? Specifications for accuracy and repeatability must be carefully evaluated for the specific applications of the machine-vision system.
- If a system works in a production environment, system specifications must be carefully defined and checked in terms of cleanliness, vibrations, temperature changes and lighting conditions.
- Careful evaluation of the machine-vision systems for their capability of measuring materials such as shiny surfaces, dark materials, and painted parts, for example.
- How fast is the inspection (parts per minute)? Will special illumination such as strobe lights be needed to ‘stop’ the motion? Will multiple systems be needed to meet the inspection rate?
- What is the cost of bad product? What is the expected ROI? As a rule of thumb, the new easy-to-use machine-vision system end up costing about $10,000 to $12,000 when you add in lighting, mechanics and encoders. This does not include design and installation time, which is an often ‘hidden’ cost if done in-house.
- What level of sensitivity to defects is needed? What levels of false accept (missed defects) and false rejects (good parts marked as bad) are acceptable? End users can’t have a perfect specification for both. The tighter the machine vision inspection specification, the more defects are found, but the more likely it is that good parts will be incorrectly rejected.
- Determine the resolution needed to make the necessary verification. This requires known parameters such as the field of view. The resolution of the system is dependent on the camera resolution vs. the field of view. The required resolution will dictate the number of pixels that cameras must have. This is also where the cost factor is important. A normal 768 by 494 pixel resolution TV format camera is priced under $1,000, whereas a 2K by 2K pixel resolution progressive scan camera will run near $10,000.
What’s next?
Despite the advancements made in machine vision technology, these new capabilities may just be the beginning. Manufacturers can expect improvements in CPU processing power that will allow for new algorithms. Improvements in cameras are also expected to continue. As the camera is able to capture a greater density of pixels, which improves image quality, finer measurements can be taken and defect detection can be improved.
And, the experts predict that machine-vision systems will be easier to use. Some companies already have systems that will allow the user to open up a box and begin using the system with an hour albeit at minimum skill levels. More plug and play and out of the box features can be expected.
“Machine-vision technology, with 3-D vision technologies at its forefront, will enter additional applications,” says Rotem. “Measurement times will be significantly accelerated, accuracy and resolution will be improved, and processes will be more and more automated, to the point where automatic classification will sort out the good and the bad parts. The ultimate and future target of machine vision will be data fed back to the process and adjustment of the production process in a closed loop. This far-reaching target, however, is still far away.”
Sidebar: Tech Tips
1. Today’s machine-vision systems are intelligent. They detect and classify defects and problems and support automated go/no-go decisions.
2. Machine-vision measurement capabilities include measurement of features such as holes, edge points, single-surface points on parts, full-surface measurement and the measurement of distances between features.
3. Machine-vision repeatability depends on the technology used in terms of data grabbing. A vision system that measures dense and large amounts of points has a higher repeatability than a system that measures only a few points.