There have been two sea changes since the widespread introduction of QMS solutions in manufacturing environment—and a third change is on its way.
In the first wave, manufacturers began to instrument and automate their facilities. They consolidated assembly procedures, removed manual and repetitive tasks, and introduced robotics. At the same time, manufacturers began to realize that their automated solutions produced data in massive amounts. Using this data at first proved challenging. Data scientists using early analytic solutions could only do so much, which meant that although manufacturers could derive insights from data, they couldn’t do this on a real-time basis or find hidden patterns.
In the second wave, third-party analytic solutions emerged. These solutions took advantage of cloud scale and speed, to provide more accurate and faster insights. On the other hand, raw data from QMS solutions was not ready for analytics. Analysts still look a long time to clean data, perform extract, transfer and load (ETL) work, and construct data warehouses. Insights came faster, but still failed to achieve improve productivity or real-time feedback.
With the past two waves, a third wave is appearing and is taking root among the best application solutions. The initial attempt on the third wave was mashing up QMS solutions with analytic solutions. These were loosely coupled and were not embedded in natural QMS tasks and activities. While this mashup may have provided dashboarding, they were not tightly coupled within the existing QMS solution.
In the third wave, users in a QMS solution will not even know they are using advanced analytic techniques and machine learning. This mashup of QMS and analytic solutions will help users be more productive and accurate in their decision making. Actionable information will appear in context to help them make better quality decisions without having to jump to a different analytic user interface. Quality investigations and decisions will ultimately be streamlined to improve the quality of their manufactured solutions.
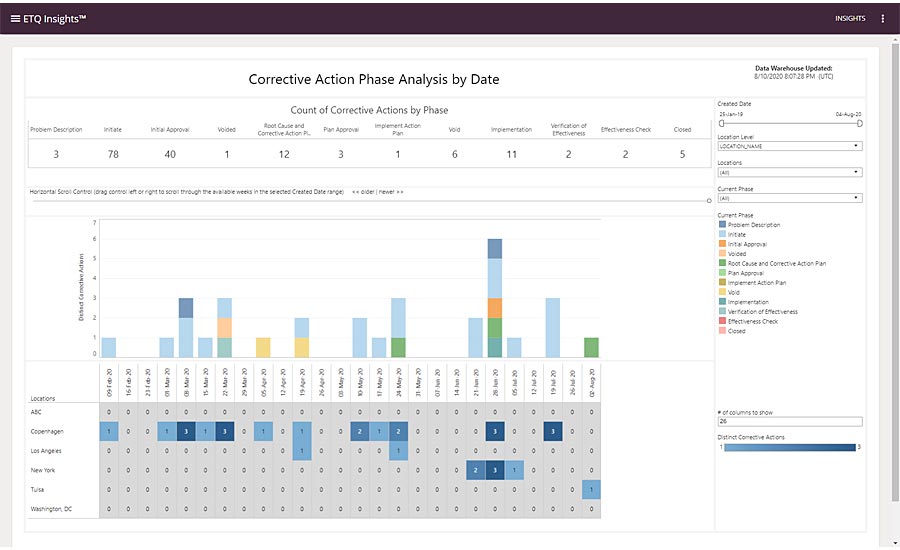
ETQ Insights’ corrective action analysis. Source: ETQ
Moving Away From The Big Picture Approach
Many industries have been taken with the idea of digital transformation, and manufacturers are no exception. Despite recent economic concerns, over 50% of companies plan to increase digital transformation spending in 2020.
By the same token, however, many companies conflate digital transformation with moonshot projects—expensive, multi-year projects that that involve efforts such as building digital twin organizations, moving systems of record to the cloud, large-scale 3D printing, and so on. Such efforts have a high failure rate. According to a study from the Harvard Business Review, only 22% of manufacturers were able to successfully scale their digital investments and achieve a high rate of return.
The paper from HBR suggests that one of the best ways to achieve a digital transformation is to start small. Create an internal innovation lab within your facility that’s charged not just with new ideas, but also the responsibility of scaling and deploying them. For best results, the teams should be cross-functional, personnel from R&D, IT, operations, facilities, and more.
As a result of this approach companies are more likely to achieve quick wins—incremental digital transformations that can rapidly improve day-to-day quality of life without vast expense or huge risks resulting from failure.
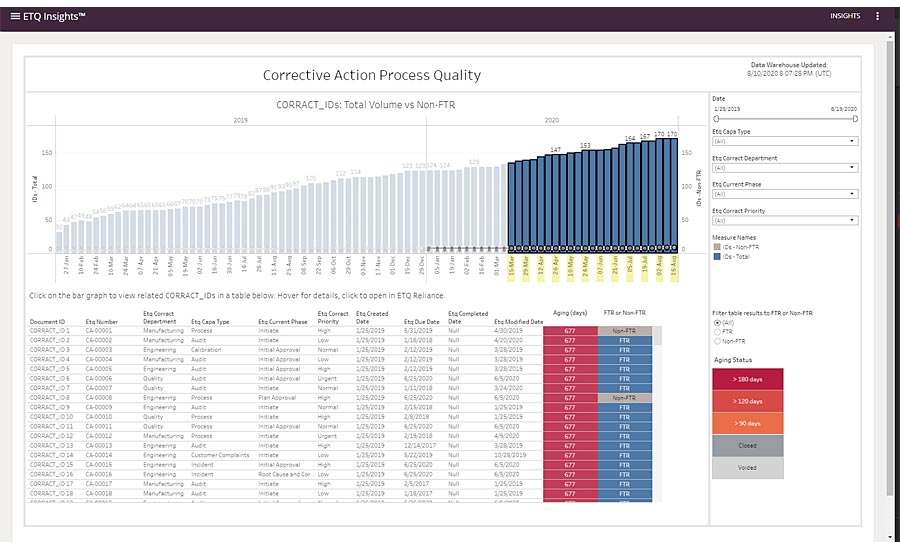
ETQ Insights’ corrective action process. Source: ETQ
On the analytics side, for example, companies still expend considerable manual efforts to track down the source of quality events such as compliance violations or nonconforming materials incidents. This effort usually entails combing through many records to find related incidents or reoccurrences that could paint to the source of the anomaly.
For a QMS solution with embedded analytics to offer an advantage over a third-party analytics solution, the QMS solution should be able to surface related events and reoccurrences in a natural and understandable way—in terms that someone who isn’t a data scientist might understand. This would allow them to assess their risks and determine the next best action in a shorter amount of time, preventing future quality defects.
To summarize, analytics vendors have been promoting moonshots at the expense of more natural use-cases using combined analytics within the QMS.
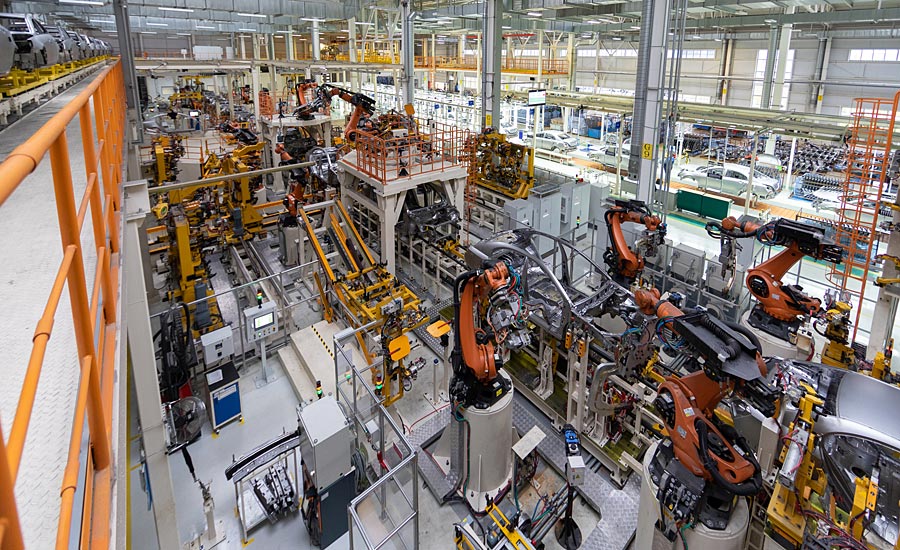
Overhead view of robots on a factory floor. Source: ETQ
Finding The Unexpected Using AI And ML
Another problem with analytics solutions—and an area where QMS vendors could add value relative to incumbents—is that traditional BI solutions only help data scientists find the things they expect to look for.
In other words, a traditional BI setup might look like a dashboard with a few graphs. One tracks nonconforming incidents over time, and another graph charts materials intake. If you look at these two graphs for long enough, there will be a spike in nonconforming incidents that coincides with intake of a particular kind of material.
The problem here is that it’s not immediately possible to tell if the two events are correlated, or if it’s just a coincidence. Furthermore, the next best action isn’t immediately apparent. Do you audit your supplier? Do you check your materials handling procedures? Do you wait and see if the same kind of material causes another incident?
In this scenario, AI and ML add value to the incident response process because they can look at more variables—more variables that can fit on a single dashboard, and more variables than any human operator (or operators) could ever monitor unassisted. This allows AI systems to find and surface what Donald Rumsfeld once referred to as “unknown unknowns” – things that are unknowable because there’s no apparent reason to look for them.
Returning to our example, let’s say that between the spike in anomalies and the correlation with materials intake, an AI system surfaces another anomaly that occurred at the same time—but was never charted on the BI dashboard. Let’s say that it was a spike in humidity that caused the material to act in an unexpected way.
Now, instead of wondering whether the original correlation was a fluke, there’s proof, borne out by a third data point, that it wasn’t. Instead of wondering about the next best course of action, there’s a clear instruction—find a way to reduce humidity in that facility. Using AI, uncertainty is replaced by clarity.
Finally, creating a QMS with embedded analytics capabilities has one last advantage for manufacturers. Many third-party analytics solutions are specialized for data scientists, but there is a cross-industry shortage of data scientists, with a vast increase in open job slots and not enough scientists to fill them.
QMS vendors have an advantage here, in that they already create products that aren’t specialized for use by data scientists. There’s a potential for them to create an analytics solution with considerable horsepower, while making it simple enough for those without formal education in data science to use and surface powerful and unexpected insights.
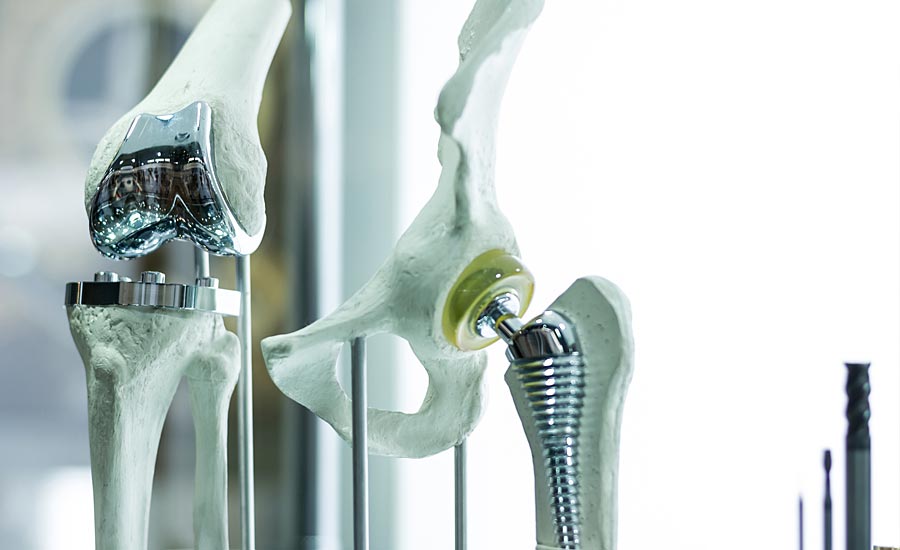
Manufacturers of high-quality medical devices can provide the body with new joints. Source: ETQ
Lessons For Using Analytics In A QMS Context
In conclusion, the best way for manufacturers to bring about a digital transformation is to architect one from the bottom up, as opposed to from the top down. Working in small groups, innovation teams can rapidly improve product quality by taking advantage of the insights offered by machine learning and artificial intelligence.
QMS vendors now have an imperative to architect and market their products along these lines. While vast transformation makes for an impressive brochure, the reality is that more prosaic efforts are the most likely to succeed. What’s more, the companies that do succeed—even if they complete transformations at a smaller scale—can still achieve improvements such as up to a 50% reduction in downtime, up to a 30% improvement in productivity, and up to a 20% decrease in the cost of quality.
These metrics mean that companies that complete successful digital transformations and properly exploit the data that is newly available to them can outcompete the rest of the industry. Therefore, QMS vendors need to seamlessly integrate analytics solutions within their software as means to make this data readily available, meaningful and actionable in order maximize the value of quality to the organization and earn their role as a trusted growth partner. Q