Artificial intelligence in manufacturing is driving quality control into the future. The race to embrace digital technologies like artificial intelligence (AI) is a critical enabler of the Fourth Industrial Revolution, such as Industry 4.0 and Industry 5.0, which will help quality control engineers in manufacturing make a great impact on our global economy.
Quality control engineers are facing a myriad of manufacturing challenges and finding it difficult to balance speeding production while still being able to deliver high-quality products to customers. The findings are that for companies to succeed, they really need to implement a digital infrastructure that positions them to fully embrace the skills and knowledge of their best assets — their people.
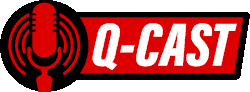
How to Use Trustworthy AI for Quality Control
As the AI landscape for manufacturers continues to evolve, it is important to get a handle on AI responsibly and what that looks like. Justin Newell, CEO of INFORM North America, explains the importance of ethical AI practices and how manufacturers can take advantage of this technology.
Listen to more Quality podcasts.
Quality control in manufacturing relies on automation just as much as on people. However, it is important to realize that manufacturing plants of the future will require at the forefront both physical and digital capabilities in order to utilize more real-time data, achieve interoperability and implement AI technology. According to recent research, 83% of top executives and decision makers agree that AI will be critical to accomplish their growth objectives.
Delivering on-time and on-budget products while maintaining high quality is incredibly important these days to manufacturers and often part of a manufacturers’ sustainability initiative. AI’s ability to improve quality control mechanisms and maximize efficiencies is real. It is already being proven that manufacturers that adopt AI early are reaping the biggest benefits. According to a recent a McKinsey analysis, expect to see significant gaps between leading manufacturers that adopt and fully integrate artificial intelligence within the first five to seven years from those manufacturers that follow suit. The analysis illustrates how AI early adopters otherwise termed “front-runners” will likely achieve a cumulative 122% cash-flow change while those that lag behind will only achieve a 10% cash-flow change. This constitutes a huge differential.
Quality Control Challenges
An engineering teams’ aim is to deliver consistent high-quality products at the lowest cost and fastest speed. Subsequently, the biggest challenges revolve around how to deliver dependably high-quality products while keeping costs low and manufacturing at a rapid pace. When applying AI-based production planning, throughput times can be reduced by up to 62% and productivity increased by up to 11% while maintaining consistent high quality, according to a recent study conducted by INFORM. These results can be achieved because such systems can take more influential variables into account better than a human ever could.
There are countless interdependencies of individual production steps for preliminary, intermediate, and final products to take into account as well as certain restrictions in terms of personnel, material, and machine capacities, which are almost impossible to keep track of concurrently. For example, ten production orders could conceivably have 3.6 million different sequences and that’s not even taking into account the number of priorities, capacities, throughput times, or other relevant planning parameters.
Even though ERPs can determine the lead time of individual orders, they aren’t able to dynamically distribute competing orders among available resources. The APS (advanced planning and scheduling) system, however, can readily calculate a sequence to determine the overall best possible outcome and generate corresponding schedules based on available resources and capacities. It can use a mathematical decision model where the AI will continuously perform a mathematical proof to show that the best solution to solve any kind of production planning problems.
Intelligent Algorithms for Better Production
Implementing intelligent algorithms deliver insights on how to make the best decisions with keeping in mind the entire production system as a whole. For example, the calculation and corresponding insight can indicate how many more rush orders could be served on time and eliminate order delays. As everyone knows, machine allocation is not just a matter of scheduling the next follow-up order on a free machine. But rather, AI could benefit the process because it could recognize more productive ways to manage orders more intelligently, safely and efficiently.
For instance, these smart algorithms can recognize up front any delivery deadlines that could require overtime (extra shifts) and could be set up to issue an alarm and deliver a clear planning forecast well in advance of any trouble. For material bottlenecks, AI-based algorithms will inform the procurement team in advance regarding which parts should be procured and by which deadlines. They identify patterns, draw conclusions, calculate lead times based on intelligence. This is much more efficient that relying on the supplier to provide the date for a particular order.
As the AI landscape for manufacturers continues to evolve, it is important to get a handle on AI responsibly and what that looks like. Manufacturing executives need to understand AI’s potential while still minimizing risks. There are guidelines that have been developed that really help to establish best practices, standards, and protocols to bringing a comprehensive approach to AI as applied in manufacturing environments while still prioritizing societal needs and individual rights.
Crucial AI Principles and Considerations
“Trustworthy AI” revolves around several transformational principles: Beneficial and human-centric AI ensures that the AI systems enrich both users and society, as well as mitigates negative impacts like bias and misinformation. It also should illustrate its supportive role to humans, enhancing decision-making processes, and upholding human responsibility. Alignment with AI is also crucial as it should guarantee AI is in sync with human and business values as well as be crystal clear and understandable when used in manufacturing environments.
For manufacturers, another principle worth mentioning is “privacy-preserving” AI, which upholds United States requirements and European Union’s GDPR standards, while achieving top-tier security standards endorsed by ISO 27001 certifications. And with reliable and safe AI standards, they should prioritize quality, consistency, and transparency within AI applications, especially in vital sectors and deliver a criteria for crafting AI algorithms that ensure safety and ward off potential threats. Therefore, adhering to “Responsible AI Guidelines” will help ensure AI benefits humanity and is transparent and ethical in nature. These guidelines arrive at a crucial juncture because of the greater AI adoption that is happening fast and furiously. It is so important that as AI grows in adoption that we as a manufacturing community make sure AI in manufacturing address more than just compliance but safeguard from risks whether with data privacy, transparency in decision-making processes or other opportunities for continuous improvement.
The truth is that AI’s potential is proving to be expansive, from industry transformation to redefining work and manufacturing processes. As research is showing that AI will surely becoming a more integral part to our lives and businesses, one foundational fact is clear; an ethical compass is essential. This is whyresponsible AI guidelineswill become a beacon for manufacturing industries and the aim today is to encourage the AI community towards conscientious innovation. Achieving trustworthy AI in manufacturing will be crucial on multiple levels for safety.