After the rapid rise in popularity of ChatGPT, and the news of it threatening the previously untouchable position of Google, many organizations have renewed their interest in artificial intelligence. After all, if you just tinker with ChatGPT, its answers are so humanlike that its interaction seems almost magical. A perfect storm has emerged consequently, with technology vendors adding AI features to their products, technology visionaries predicting a profound impact of this technology on our society, and buyers left scratching their heads how to make sense of it all.
In the quality management domain, AI undoubtedly has potential in different areas. And if you think about the longer-term ramifications of this technology, it would be easy to think that AI could be a threat to less modern tools like statistical process control. Or even worse, that it will render SPC obsolete. This article aims to demystify some of the use cases of AI.
Not All AI Is The Same
First, let’s understand the difference between generative AI and predictive AI. Simply put, generative AI (such as ChatGPT) lets computers create content (from texts to deepfake videos or images). Those humanlike conversations are a good example of generative AI. On the other hand, predictive AI analyzes existing data. A good example of predictive AI is machine vision, where camera images of a production line are analyzed and used to check the quality of produced goods.
Both predictive and generative AI have potential applications within quality management. While the most recent hype around ChatGPT has been about generative AI, the most obvious use of AI in quality management is predictive AI.
I would argue that predictive AI has been used in manufacturing for almost a century when Walter Shewhart started doing statistical analysis of quality control data. After all, SPC and predictive AI both apply statistical algorithms on large datasets. If there ever has been a moment for quality people to make a claim to fame it is now! This is a gross simplification of the two concepts of course; while both use statistical analysis, SPC does not include machine learning, i.e. the capability of the algorithms to learn and adapt their outcomes based on newer data. However, SPC in manufacturing is hard to beat, as it has matured for almost 100 years, and has been tried and tested in many manufacturing scenarios.
Should You Opt For Modern Tech?
The main thing that sets SPC and AI apart is:
- SPC highlights the things you know you don’t know
- AI has the potential to highlight the things you don’t know that you don’t know
Are you still with me? In short, predictive AI has the potential to detect patterns outside of the predefined SPC patterns. More specifically, SPC tends to look at variations in one data attribute, such as color, pH, or weight. Predictive AI can identify correlations between different data attributes, and thus predict previously unknown deviations based on the combination of color, pH, AND weight. In addition, it can look at correlations between quality control (QC) data and other data sources. For example, you can use QC data combined with data about machine maintenance to predict maintenance required in production machinery. An even more ambitious way would be to combine structured QC with unstructured data, such as customer complaints. An interesting example is one in which researchers were able to predict required production volumes of a Dutch beer brewer, based on consumers’ comments on social media. Pretty wild!
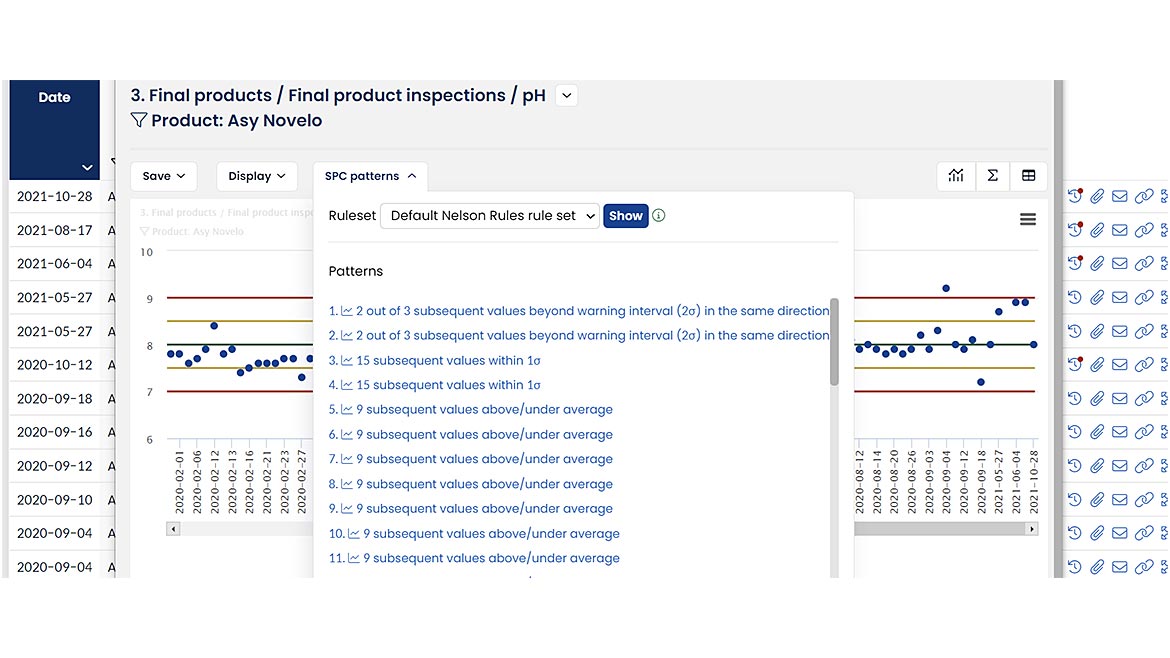
AI Is Not A Quick Fix!
Tantalizing as these new opportunities may seem, AI is not a quick fix! There are some critical prerequisites to the successful usage of predictive AI.
First of all, access to large sets of data is key to getting value from AI. Many manufacturers simply don’t have those data sets, as data is scattered across many different systems in different formats ranging from paper to Excel to databases. More mature organizations have started creating data lakes that gather many different types of data in one location for analysis purposes. However, creating these data lakes is both a big organizational and IT challenge. Questions like ‘what data do we gather,’ ‘how do we curate this data’ and ‘how do we aggregate this data’ all require thoughtful consideration.
In addition, creating data lakes requires a lot of technical knowledge. And applying AI algorithms to this data is also a highly specialized task. This leads us to our second prerequisite: access to skilled resources that master AI and can apply it to your business in a sensible way. Where SPC can apply the same algorithms to any data attribute, predictive AI actually needs to be trained to look into specific problems. To train the algorithm, you need data scientists that understand AI and know how to apply it to a specific problem. Data scientists however are a scarce resource these days: with the U.S. Bureau of Labor Statistics projecting a growth rate of nearly 28% in the number of jobs requiring data science skills by 2026. And it becomes even more challenging since many young professionals in that labor pool prefer to work in environments other than manufacturing.
This leads us to our third prerequisite: we need to be able to trust the outcomes of predictive AI. AI answers aren’t always reliable. So how do we know when they are reliable or not? There is a lot of focus on this problem, and reliability will have to improve before AI can take a central role in enterprises. And how do we know that these predictions make sense if we don’t understand the underlying algorithms? Search volumes for SPC related terms on Google are high, which indicates that many people still struggle to understand even these 100-year-old algorithms, let alone the much more complex AI algorithms. As a consequence, investments in AI have unsure outcomes. Research by Gartner shows that while 69% of quality leaders are actively using or piloting predictive analytics, only 17% are confident about effectively implementing the technology.
While it’s great that so many manufacturers are experimenting with Predictive AI, it’s essential to not be fooled by the current hype around AI. Which leads to our fourth and last prerequisite: you need to know which problem you are looking to solve with AI. Starting with the technology and trying to find a problem to solve can lead towards success. But it ends in failure much more frequently. As with any new tool, learning how to master it and when to apply it requires skills, knowledge, and experience. As an avid DYI enthusiast, I can say that as soon as you get a new tool, you are bound to use it more often than makes sense. Only after using and misusing it for a while, you learn the best use cases for its application.
So, Will AI Kill SPC? Should We Replace SPC With Modern Tech?
My answer to these questions is a resounding NO! SPC has proven its worth and will continue to be relevant in most manufacturing scenarios. That doesn’t mean manufacturers shouldn’t look at AI initiatives. Over time, AI will add a valuable stream of different insights that will augment and complement traditional QC and SPC insights. Finally, let’s look at some guidelines for the adoption of AI within manufacturing.
- Take your digital transformation seriously. Without digital data there is no use for AI, so start looking at initiatives to gather data. IIoT and edge computing are becoming more accessible and affordable and are great tools for digitization.
- Leverage the existing technologies you have, such as ERP systems, QMS software and MES software. Just by using the data already collected in those systems and taking it out of their silos you can generate new insights without having to adopt AI technology. You will need to learn how to do this anyhow for your AI projects later.
- Use your existing SPC algorithms on wider data sets. Again, SPC has proven its value, so even looking at data sets outside of the traditional QC domain can already give you some great insights!
- Focus on your problem areas first and understand if AI is a potential solution to those problems, not the other way around. Is AI a better solution? Or should you consider other technologies?
- If anything, experiment with some of the proven AI use cases, like machine vision or predictive maintenance.
- Start building your skills both in business as well as in IT. Start with creating cross-functional teams to learn how to be methodical about data gathering and understanding what data provides value to your business. For example, start experimenting with understanding non-structured data such as customer complaints, and how to combine this with traditional QC data.
In short: think of AI as just one element of your roadmap towards Industry 4.0 initiatives and make sure you prioritize accordingly.