You can’t escape the buzzwords. The Internet of Things. The Industrial Internet of Things. Edge Computing. Industry 4.0. Big. Data. Every practitioner has their own, slightly different definition of what these terms mean—a fuzzy cloud of vague meaning. I’d like to point out a gaping hole that most of these definitions miss and shift how you think about the connected technologies you use as a quality practitioner. If you take away one thing, I hope it’s this: it’s the combination of people with the Internet of Things that creates a holistic solution for the shop floor.
Since before computer time began, the internet was about connecting “things”: servers to servers, servers to computers, and the fundamentals of the backbone technologies which make it all possible have remained largely unchanged. What has changed—and at a relentless pace—has been the exponential increase in computing power and the exponential decrease in its cost. Though the “Internet of Things” itself may be a misnomer, IoT is driven by this macro trend, and with computing devices smaller and less expensive to deploy, hardware and software makers are searching for new uses.
On the factory floor, this means connecting machines to gather performance and sensor data. Measuring KPIs such as Overall Equipment Efficiency (OEE) became fantastically easier because a large portion of the data required to drive the stats are gathered automatically using cheap and available sensors. OEE is easy to track and is a rough proxy for operational efficiency. With an easy measurement like OEE, the actions of engineering and management to improve quality and efficiency can be reflected with a thumbs-up or thumbs-down score. Problem solved. These simple metrics have been so successful, there is now a cottage industry of vendors with machine monitoring applications of varying levels of sophistication.
But what about the human element in manufacturing? How do you measure Overall Process Efficiency (OPE), which incorporates both machines and people? How do you know whether the shift changeover procedure was adhered to? Did the operator properly apply thermal paste? Was the O-ring correctly placed? Who on the white team is most consistently producing quality goods, and is there a time-cost for her good performance? The traditional perspective on the Internet of Things, focusing only on machines, cannot answer these questions.
Even at the most automated facilities human operators are responsible for correctly configuring, maintaining and monitoring machines. Now that we can easily gather lots of data from machines, we can monitor them closely and predict problems before they happen, right? Isn’t that the promise of Industry 4.0? Maybe. Machine data isn’t useful if you don’t have a good way for a human operator to take action based on the machine’s data and you have a way to verify that the process has been done correctly. Moreover, you need traceability over those human operations whether for compliance reasons or just better operational management. Unlike machines, human operators don’t have convenient communication interfaces that allows us to understand what is being done and what mistakes arise. While manufacturers have increasingly greater visibility into their machines through IoT, the human element within manufacturing environments remains a black box.
Unlike IoT-focused platforms, the legacy quality management systems (QMS) we use in the factory take a more operator-centric approach, but they also suffer from rigid workflows that are difficult to customize to real-life shop floor scenarios. They offer interfaces that are siloed, not user-friendly, and lack the ability to integrate simply (and cheaply) with machines, sensors and tools. The truth is that quality is a moving target that requires a flexible software platform able to adapt to an in-flux factory environment. IT-led custom development projects can result in a quality system that’s tailored to a factory’s unique challenges but they tend to be expensive and extremely time consuming to deploy. Their bespoke nature makes them difficult to maintain and upgrade.
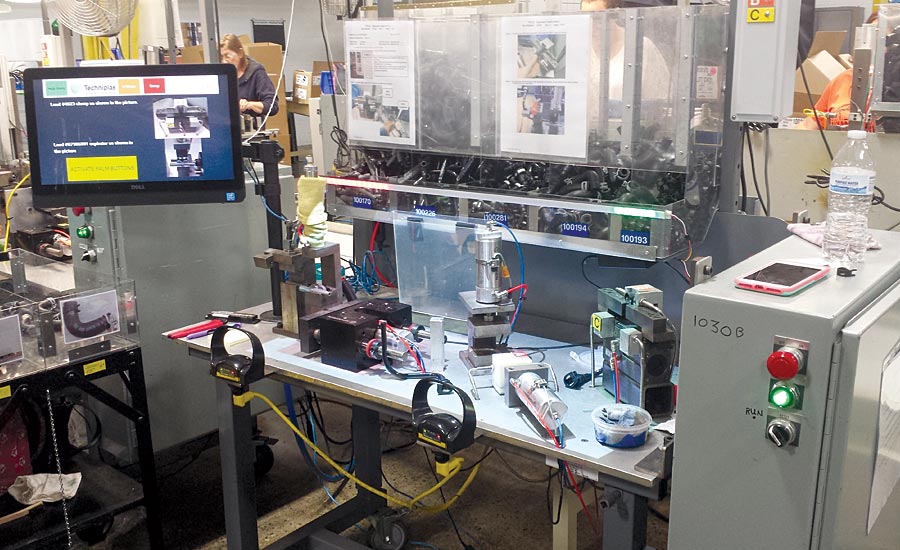
Tulip powers the manifold assembly area at Techniplas. This is a Techniplas assembly station.
Next Generation Quality Tools
Peeking at the future, I want to share a couple of examples where shop floor IoT is incorporated in a flexible, quality-integrated and human-centered software system.
At Techniplas, an injection molding company, a manual assembly workstation was outfitted with a manufacturing app integrated with sensors to help guide operators through the semi-automated process of assembling hoses to manifolds. The configuration of the parts at the station are numerous, and each variant is visually similar. Moreover, insufficient supply pressure to the assembly machine could lead to assembled parts that looked okay, but which failed downstream QC. Techniplas’ solution was integrated: A small IoT gateway device was connected to a pressure sensor and a simple pick-to-light system to pick the correct part. These in turn were connected to a PC providing the operator interactive SOPs. As assembly progresses, the pick-to-light system illuminates the correct bin of parts and the sensor ensures that the pneumatic system is in-spec. If the system gets out of spec, the supervisor is called, and the operator receives a visual warning. Production data is collected through the built-in analytics engine and is available in real time for line supervisors. Net result: zero defects at the station.
“Production has gone up and we reduced defects to zero,” said Shannon Nichols, manufacturing engineer at Techniplas. “The system collects data continuously and with the built-in analytics we are able to see the timing per step for each and every part in order to identify the bottleneck and concentrate on that individual step. It has also allowed us to train operators using the system, as opposed to having to utilize another operator for training. As the engineer responsible for the line, I am in total control of the manufacturing app and can customize it, adding operator workflows and sensor integration without writing any code.”
Another example of the future of quality systems can be seen at Dentsply Sirona, a company whose comprehensive dental solutions include product brands across consumables, equipment, technology and specialty products. Dentsply Sirona’s Implant division provides solutions for all phases of implant dentistry, including the design and manufacture of patient-specific, implant-supported abutments and crowns through its Atlantis portfolio.
Because Atlantis solutions are as unique as each individual patient for which they are designed, every product package contains a varying combination of components, such as screws and insertion guides, which the clinician will need to install the final dental implant-supported restoration. Every package is one-of-a-kind; and there are hundreds of thousands of component combinations. Obviously, it is critical to get the right components in the right box.
To successfully achieve this consistent accuracy in packaging and delivery, Dentsply Sirona Implants used the manufacturing app platform to design and deploy a quality app that powers its product verification station and completely guides operators through this complex process. A manufacturing app running on a standard computer displays simple instructions with a complex back end system that talks directly with Dentsply Sirona Implants’ ERP system. At the same time, an integrated pick-to-light system illuminates bins of components. The whole validated process dynamically guides the operator through the packing sequence, reducing the risk of making a mistake when preparing an order. Overall quality outcomes are no longer solely dependent on the operators. This enables standardized workflow, reduces training time for new operators, increases throughput, and yields better quality outcomes.
“The system allows us to control a highly complex process with hundreds of thousands of variations while leveraging the investment we made in our existing backend systems,” says Dan Ron, Dentsply Sirona Implant’s senior process engineer. “The system is self-serviced so we are able to customize the manufacturing apps to our needs, which allows us to solve our own problems and reduce our dependency on external vendors and better focus our IT resources. Today, we are capable of having real data with real evidence from our process that helps our staff have more visibility of process efficiency, while allowing us to effectively manage our patient-specific components for each and every order.”
Both these systems combine interactive work instructions—the human element—with sensor-based error-proofing, but there are simple systems that can have large impact. New Balance’s challenge was that quality was a very manual process. Data collection using paper was difficult. Additionally, it was hard to make all the collected data actionable and to share the information on defects between one production line and the next, let alone between factories. In their domestic factories, New Balance implemented a quality software system that optimizes for operator ease-of-use and the collection of rich data using tablets and touch-screen PCs distributed throughout their work streams. At quality checkpoints, skilled operators report defects and their classifications just by pressing on an image of the shoe where the problem exists. This simple digital “form” replaces forms-on-clipboards that generated reams of paper that had to be manually entered and tabulated on a computer. “With the system, we are now able to capture all this real-time data and get visibility into the root causes of production issues,” says Edith Harmon, vice president manufacturing innovation, New Balance. “We now have a lot more confidence in the data, and we can digitize the analysis. We can feed it all the way back to the designer. We can use the data to track production factory to factory and value stream to value stream. We can also digitize our lean practices and promote standardized work throughout our facilities to better measure quality and performance.”
What does the future hold?
The commonality between the quality software systems in these stories is their use of self-service manufacturing apps that allow process engineers to create and maintain operator-centric quality workflows that are completely customized to their specific manufacturing processes and the plug and play integration of shop floor IoT to track and verify operator workflows. They each designed quality directly into the process using technology appropriate for the task—in some cases, it’s as simple as an off-the-shelf tablet. In others, these interfaces are a dance between multiple sensors and operator interfaces that provide direct feedback. This is the incredible opportunity for the so-called “Internet of Things” for manufacturers: forging that last-mile connection between the people on the factory floor, the tools, machines and sensors they use, and the procedures they execute.
1 January 1, 1970, for the geeks out there. Computers typically represent time as the number of seconds since some known reference time-point. For most computers, this date is January 1, 1970.
2 https://www.internetsociety.org/internet/what-internet/history-internet/brief-history-internet