The production of battery cells is a complex process that relies on extreme care and precision so that the batteries meet the necessary safety, performance, and durability standards. For quality assurance purposes and to optimize the manufacturing workflow, machine vision is employed throughout the entire process to identify production errors, damage, or impurities early on.
The McKinsey consulting firm expects that the entire process chain of lithium-ion battery production, from extracting the materials to recycling, will reach a value of more than 400 billion dollars by 2030. Cell production accounts for around 30 percent of the value created. This process is divided into three steps: electrode production, cell assembly, and formation and aging. All three steps in the process require maximum precision, which also means a high potential for error that can be reduced by means of machine vision.
What is machine vision?
Over the past decades, machine vision has increasingly established itself as an indispensable technology for companies in a wide range of industries. As the “eye of production,” machine vision always keeps an eye on all processes related to manufacturing and logistics. This requires a hardware and a software component: So-called image acquisition devices act as the former. These can be cameras or sensors positioned at various points in the production environment. The devices seamlessly record everything and generate large amounts of digital image data in the process.
This image data then must be processed to be useable. This is done by machine vision software that is connected to the image acquisition devices. By processing the provided visual information, the software can detect and analyze patterns, shapes, colors, and other attributes in real-time, facilitating tasks like object recognition, defect inspection, and even complex decision-making based on visual input. As a result, machine vision enhances automation, efficiency, and precision across various industries, transforming how machines interact with and understand the visual world.
The technology is indispensable for modern, highly automated inspection processes as part of operational quality assurance. It can be used to reliably detect any type of defect in objects. The faulty products can then be automatically classified as rejects and sorted out before they enter the further process chain. In addition, objects can be measured precisely to ensure the correct dimensions and thus the required product quality.
Modern machine vision solutions can benefit companies in several ways: For example, the productivity of the entire process chain, from assembly to defect inspection to logistic workflows, is raised to a new level. In addition, costs can be saved on a large scale through leaner and highly automated production processes. And finally, customer satisfaction and trust are secured in the long term through optimized product quality.
Machine vision in electrode manufacturing
Product quality is an essential part in every step of the mass production of batteries. For the manufacturing of electrodes, copper or aluminum foil is coated on both sides with an active material. The coating must be within a narrow tolerance range and be precisely aligned on both sides. Any impurities, inconsistencies, or defects will impair the quality and performance of the entire battery. Machine vision is used to check the surface, dimensional accuracy, and orientation of the coated electrode sheets in order to ensure their quality (see Figure 1). Along with dedicated quality control, results delivered by the machine vision software permit consistent process optimization, sometimes in combination with other data points. This makes it possible to determine early on whether, for example, the coating was not applied uniformly, the width of the electrodes is inconsistent, or coating residue adheres to the edges (see Figure 2). What makes this more difficult is that the coating process takes place at high speeds of up to 80 meters per minute and with a coil width of up to 1.5 meters. Machine vision and the corresponding hardware (such as line scan cameras) can robustly detect and classify the smallest defects even under these production conditions.
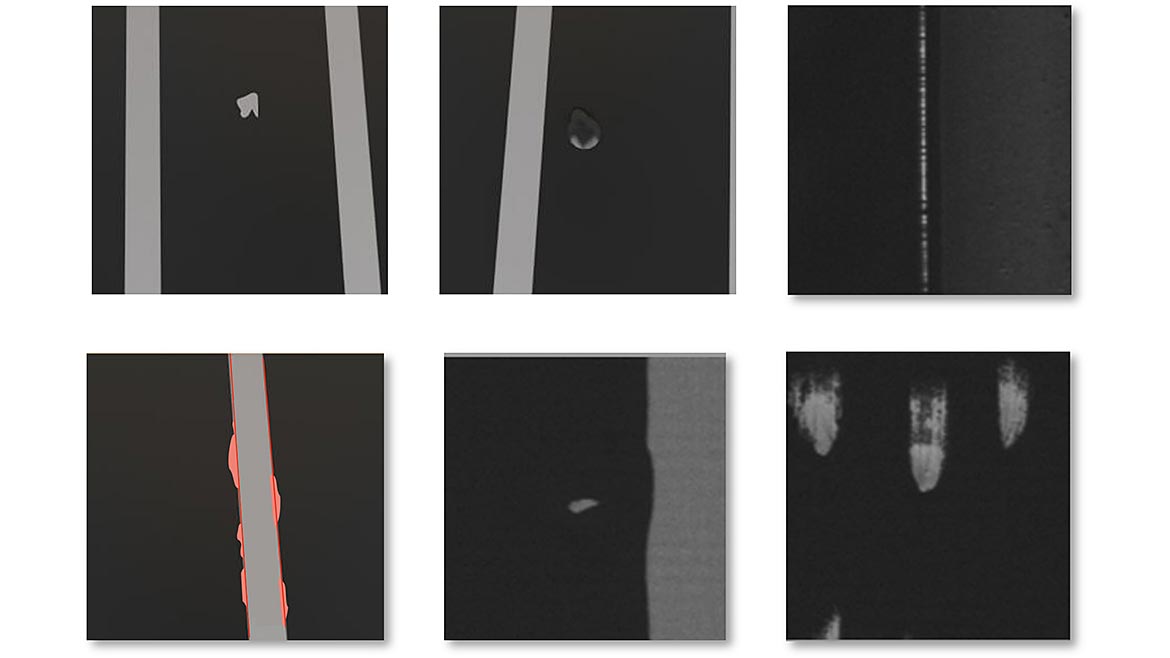
In the separation steps (slitting, cutting) that follow, and depending on the battery cell format, the coated electrode foil is separated into narrower strips or individual electrode sheets that have the desired dimensions for subsequent cell assembly. This process also requires maximum precision. However, not only does machine vision check dimensions, but the software also detects burrs, impurities, cracks, and unclean cuts.
In electrode manufacturing, there are a number of applications in which machine vision, along with surface inspection and measuring, is essential for precise orientation and positioning. To detect defects of any type and size, methods for recognizing anomalies can also be used to identify hard-to-define defects. When inspecting the surface of the coated electrode foil, powerful machine vision software can robustly reveal defects and impurities even under problematic contrast conditions. It also recognizes the orientation and positioning of coated surfaces, even in low-resolution images.
Avoiding errors in cell assembly
The next production step after electrode manufacturing is cell assembly, where the electrode foils and a separator are first stacked (prismatic or pouch cells) or wound (cylindrical cells) and then inserted into the battery housing. Most of the housing is subsequently welded and filled with electrolyte via a remaining opening. Once the filling process is completed, the last opening is also closed. Once again, machine vision software is used to check the individual work steps to assure the necessary quality.
The weld seams can also be inspected in this way. However, due to how the welding process takes place, a wide variety of different defects can occur, which is why AI approaches are well-suited (see figure 3). In contrast to an appropriately trained deep learning network, the human eye would not be able to detect and quantify patterns or make decisions based on these patterns. Furthermore, thanks to machine vision, bar codes and Data Matrix codes can be read robustly, even during motion and changing lighting conditions.
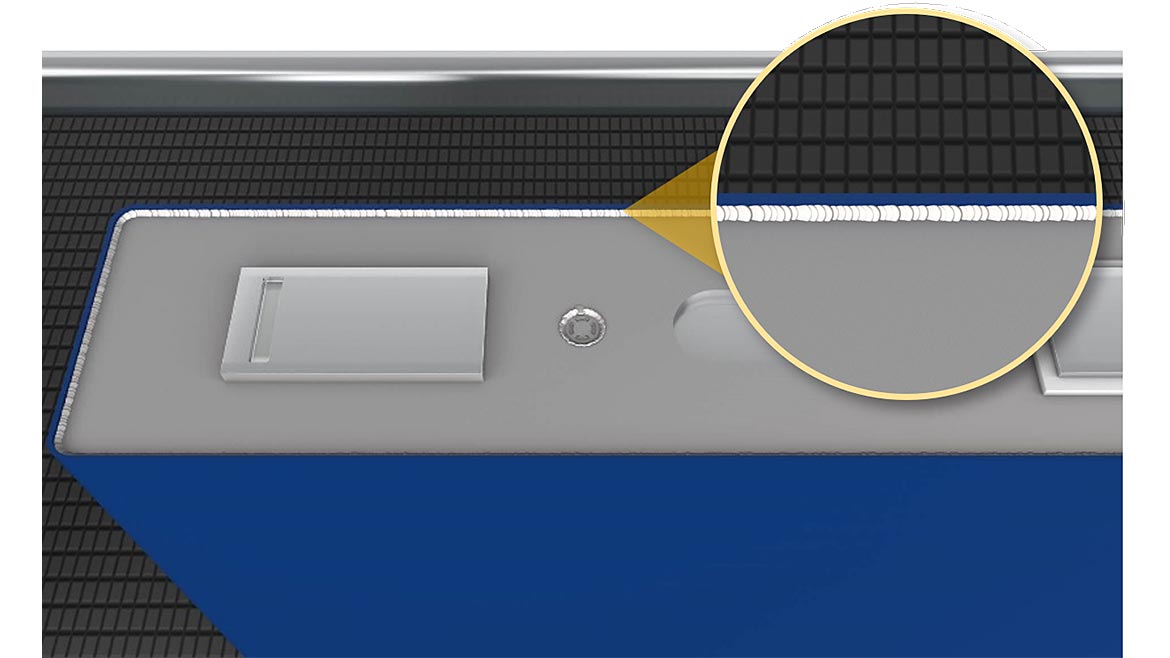
End-of-line tests prior to shipping
Vision & Sensors
A Quality Special Section
Before the battery cells leave the factory, they undergo a variety of end-of-line tests, including visual inspections. After the tests, the cells are sorted (usually according to their power capacity), packaged, and prepared for shipping. For visual surface inspections and measurements, machine vision allows for quickly determining whether cells need to be rejected. If a cell is deformed, deviates from the specified cell diameter, or has any other type of surface damage, the machine vision software classifies it accordingly. Unreadable codes, impurities, or foreign bodies can also be robustly detected. In this way, cells classified as damaged can be rejected before they leave the factory. Machine vision can also identify damage on highly reflective surfaces easily and automatically, including small scratches, dents, or bulges.
Methods applicable to cell production
As illustrated in the paragraphs above, battery cell production is divided into numerous individual steps where machine vision enables 100 percent inline quality control and significantly contributes to process optimization. Both classic, rule-based methods and deep-learning-based methods are employed. The former are mainly suitable for very fast production steps, such as coating and calendaring, or when maximum precision and dimensional accuracy are required – for example, when stacking individual electrode sheets during the production of prismatic battery cells.
On the other hand, deep learning technologies increasingly come into play in the later stages of cell production, particularly for defect detection and surface inspection. These technologies permit a robust detection of the smallest defects and contaminations, even on highly reflective surfaces. In addition, the deep learning technology known as anomaly detection allows previously unknown defects (anomalies) to be identified. Another advantage of this method is that only “good images” are needed to train the model.
Machine vision makes battery production sustainable and competitive
The use of machine vision in battery production offers numerous benefits for manufacturers. The consistent integration of machine vision as part of a comprehensive digitalization and integrated process optimization strategy holds significant potential. It enables a reduction in reject rates and makes a measurable contribution to sustainability thanks to the more efficient use of valuable raw materials. At the same time, it increases output because, as the “eye of production,” machine vision facilitates fully automated and seamlessly traceable production processes.