It learns like us humans, supports us in our everyday lives and yet it scares us: artificial intelligence. It is gradually finding its way into industry. But what about quality assurance? What can AI already manage, what is (still) a dream of the future and how much responsibility do we actually want to give it?
The development of AI
Today, artificial neurons use algorithms to imitate the nerve cells in the human brain. These complex connections – the neural network – enable machines to solve tasks from different areas. The neural network learns independently and can improve itself. The basis of all artificial intelligence is therefore the self-learning algorithm. However, this is preceded by a number of necessary developments. Improved computing power and large amounts of data are prerequisites for machine learning to be possible at all.
The training
The training of the AI is very extensive, especially in terms of the amount of data required. The AI itself knows nothing and can do nothing. Unlike humans, it does not perceive differences in color and size and allocate accordingly. What seems to happen automatically in humans when they are babies, AI has to be taught in minute detail. However, since AI, unlike humans, is highly specialized, it does a very good job with simple processes that require a great deal of concentration from us humans. This naturally also reduces its susceptibility to errors. A well-trained AI is not only more accurate, but also faster than people are.
Artificial intelligence, machine learning and deep learning
Any technology that enables computers to work with logic and rules is considered AI, whereas we speak of machine learning when the computer itself learns from its experiences and can draw conclusions. Deep learning, on the other hand, refers to the creation of a neural network as described above, which is a sub-area of machine learning. This neural network has several hidden layers that process different impulses in order to produce a specific result.
What is already possible today
In measurement technology, AI is still in its infancy. Its main field of activity is very much limited to image processing. Two major task packages are currently available for evaluation: Segmentation and classification:
Segmentation |
Classification |
As the name suggests, the AI breaks down those regions that are of interest for quality assurance. |
Classification is an allocation according to properties. For example, the AI can name the laser class of a laser-treated tool or the object type in general. |
The training works the same for both tasks. You provide the AI with labeled data. Let’s take a “dot” or a “scratch” as a metrology example. The data sets you feed the AI with already contain the corresponding label “dot” or “scratch.” As a result, the AI learns what a “dot” is and what a “scratch” is and can subsequently evaluate independently: “This is a scratch.”
The risks of AI in measurement technology
The risks in measurement technology are basically the same as in any other field that already works with AI. We have insight into the neurons that we feed and we see how the areas of the neural network that deliver results work. What happens in between is really a black box for us humans. So how exactly the algorithm learns is not tangible. In measurement technology - and let’s actually stick to the two classic work steps of segmentation and classification - this also means that we have to trust fairly blindly. Of course, this has to be taken into account when we talk about the decreasing susceptibility to errors, as mentioned above.
Will AI replace us?
Since we are already talking about the fact that AI could relieve the burden on measurement technicians, we must also ask ourselves whether artificial intelligence will replace us in quality assurance. From today’s point of view, this is unlikely. One of the biggest challenges at the moment is finding qualified personnel in the first place. The shortage of skilled workers does not stop at quality assurance. Even simple tasks require very precise work. On the one hand, AI would intervene in an area that is already difficult to fill and, on the other, we would relieve employees of all the tedious detail work, which allows us humans to concentrate on more important things. This leaves more manpower for more complex and valuable issues. We can therefore strengthen the effectiveness of employees through the use of AI.
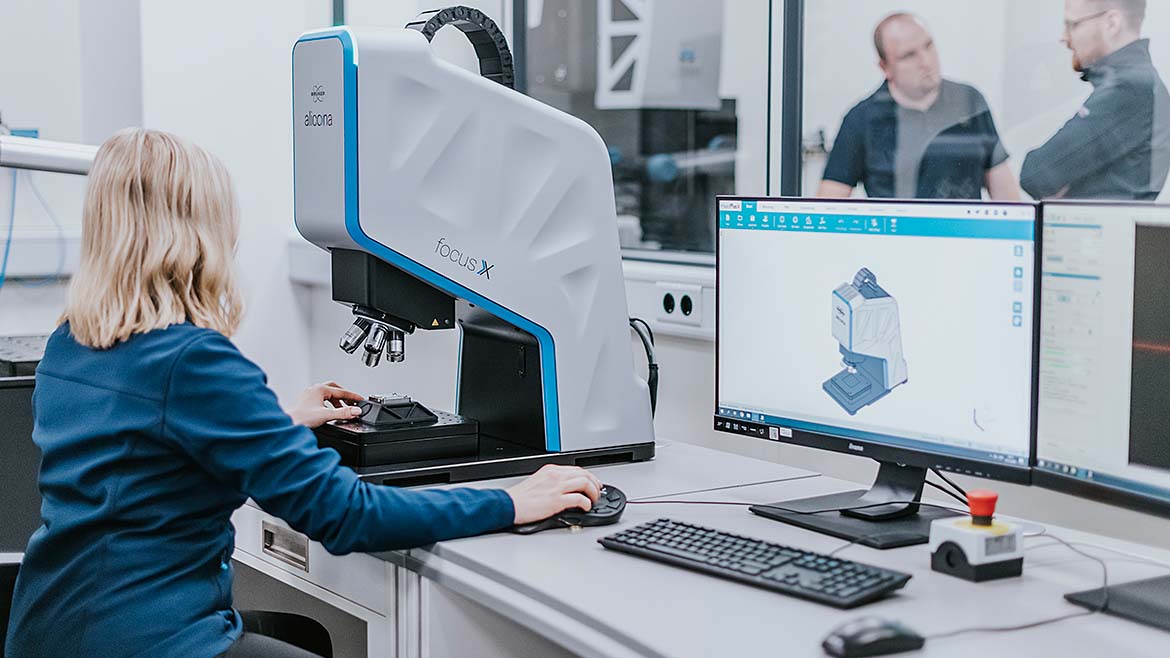
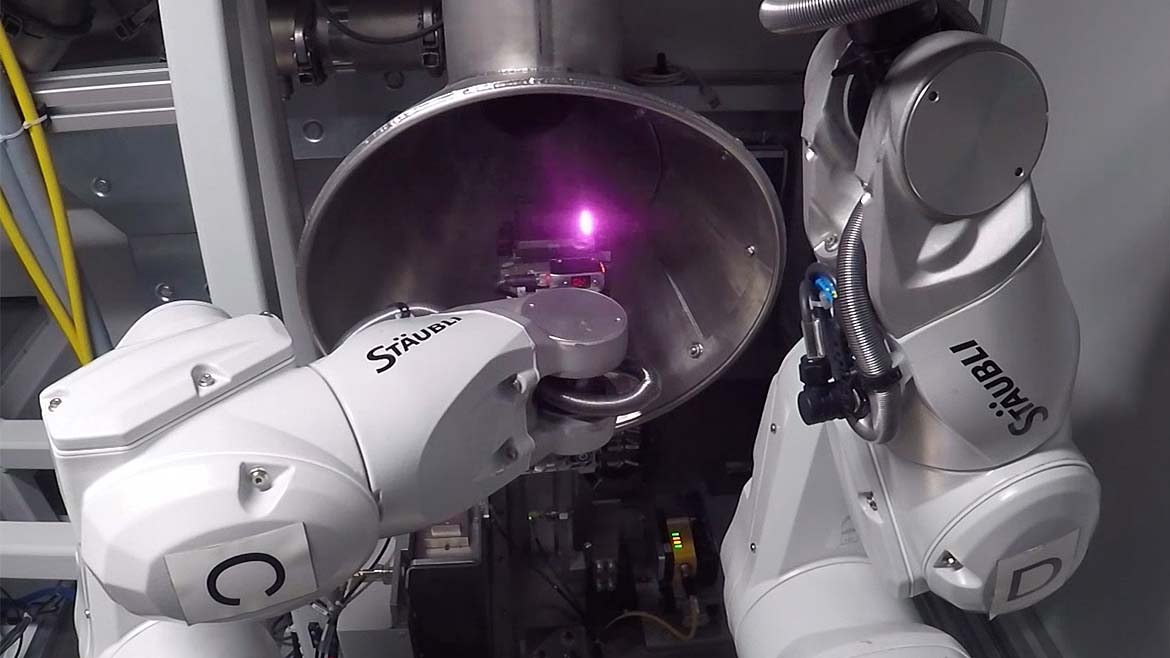
Let’s get specific about AI in optical metrology
Some optical measurement device manufacturers use artificial intelligence in three areas already:
- AI is used internally to improve and further develop the company’s technologies. This involves optimizing the algorithms for instance to better determine the focus points, eliminate measurement noise and so on.
- Customers are provided with measurement data so that they can train the artificial intelligence. This service is important due to the extremely large amount of data required to create the algorithm.
- The development of an artificial intelligence solution specifically for the customer. For this purpose, software is programmed using deep learning.
Optical measurement technology is far ahead of tactile technology when it comes to AI applications. The areal surface measurement in particular, which only an optical measuring device can provide, is worth its weight in gold. But like everything in the field of AI, the full potential has not yet been exploited.
Applications of AI in measurement technology
- Defects
- Segmentation of laser class
- Grinding grains
- Position and orientation
- Shot peening
- Contour
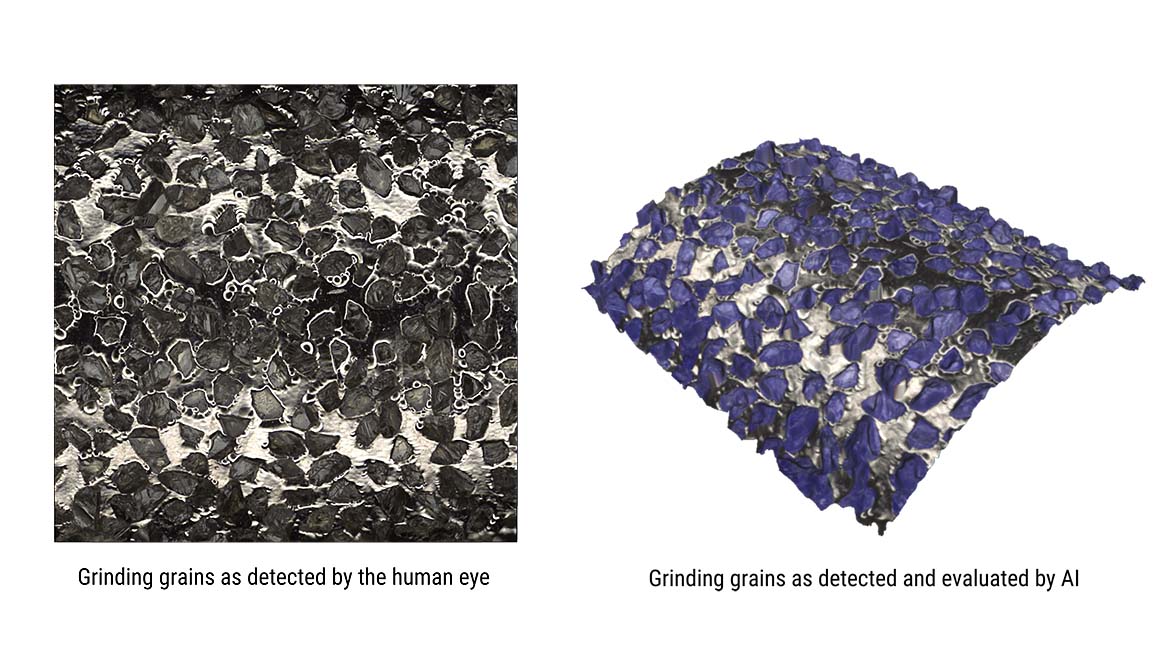
Practical example: Surface finish of a grinding pin
Figure 1 shows all the fine abrasive grains of a grinding tool. And what do you usually do with them? Estimate how big, deep, wide, distributed and so on they are. This is a perfect application example for AI. At the top, you can see the surface of the tool as the human eye perceives it. At the bottom, you can see all the abrasive grains that the artificial intelligence has found. These are the blue dots. The AI makes any estimation obsolete because it can produce an exact result - completely effortlessly compared to humans. Of course, the AI has been shown in advance what is and is not a grinding grain. It receives data from which it learns to identify such grains. This enables it to evaluate not only the quantity but also the distribution, height and so on. This can be seen in Figures 2 and 3. With conventional image processing methods, it is difficult to program a generally robust solution. There are many different grain materials and binding materials and also many different geometries, all of which lead to difficult lighting conditions. But AI can cover this quite well.
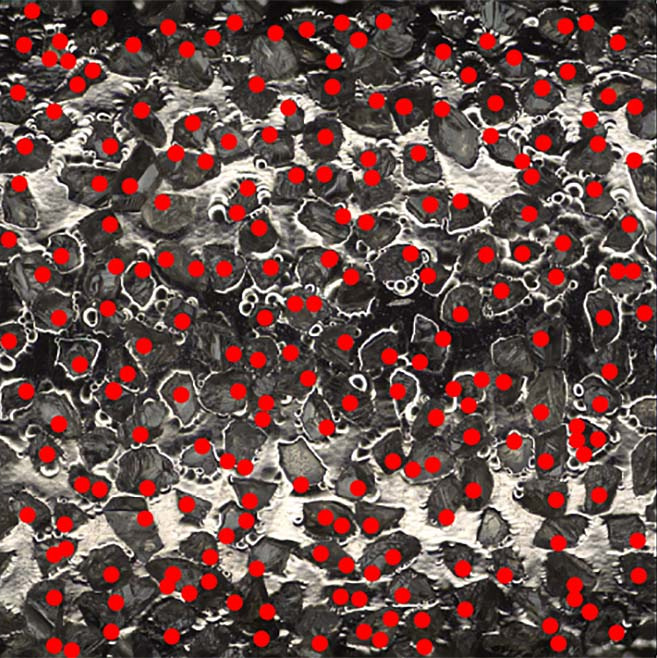
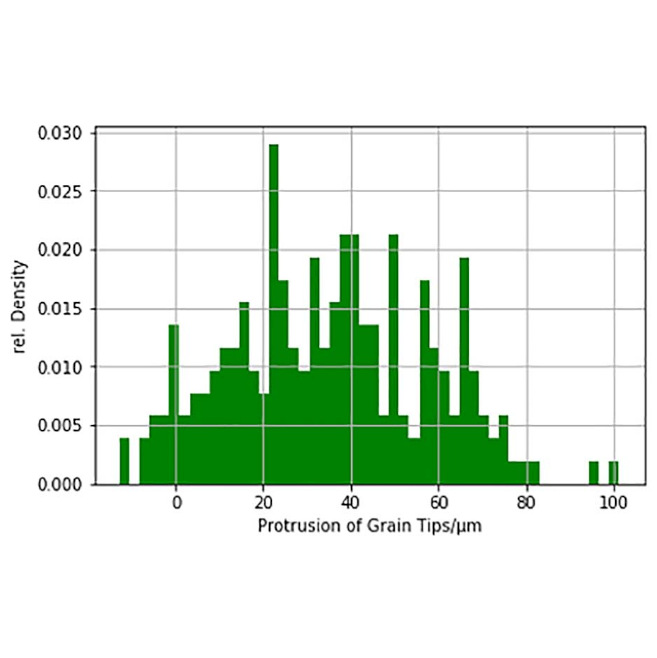
Where is AI heading in measurement technology?
Opinions differ as to whether AI will revolutionize measurement technology. While some industry representatives believe that AI will never be able to do more than image processing, others think that it has much more potential than simple segmentation and classification tasks.
Future scenarios
When we talk about further development steps, we need to think beyond the digital twin and recognize the need in all processes. Smart creation of measurement plans is conceivable, although some industry experts are skeptical about this development. With the help of CAD data and PMIs, processes could be self-learning or at least greatly simplified for the measurement technician. Let’s go one step further and place the AI in a control position that communicates between metrology and production. This could mean that the AI not only recognizes defects but also draws conclusions about deficits in production. In turn, it could independently report these back or even initiate the corresponding adjustments in the production process. All of this is conceivable. However, whether and when AI will develop to this point is still up in the air.