Within the realm of industrial automation today, few standalone technologies exist. Artificial intelligence (AI) is a prime example, as AI does not represent a single technology, but rather a set of multiple technologies and techniques including machine learning, deep learning, and machine vision.
AI-enabled software has become increasingly popular — and an increasingly common buzzword — in industrial automation, but unlocking the true potential of its power involves integrating technologies such as traditional machine vision and robotics to work in synergy with AI algorithms to accomplish disparate tasks. For example, with technology today, it is possible for automated visual inspection systems to leverage a range of different technologies to optimize a plan for image acquisition and lighting parameters to ensure that critical defects are found by the system. When properly designed and implemented from application feasibility analysis to final system validation, such systems can add significant value on the plant floor.
Productivity Despite Uncertainty
Despite some slowdown in robot installations in 2023, the International Federation of Robotics’ World Robotics 2023 report shows that a record number of 600,000 units are predicted to be installed in 2024. Market intelligence company Interact Analysis offers a similarly optimistic outlook, forecasting 5–7% growth in the industrial robot market out to 2027.
In terms of AI adoption in manufacturing, the Future of Industrial AI In Manufacturing survey from the Manufacturing Leadership Council shows that 57% of respondents are experimenting with a range of small-scale pilot projects while 28% are implementing projects operationally that have passed the pilot stage. Out of all respondents, only 4% have no plans at all to implement AI into their manufacturing processes. Key application areas for AI technologies named in the survey include quality improvement (30%), vision systems (21%), and robotics (20%).
Increasingly, more industries around the world rely on automation technologies to drive productivity and throughput during an ongoing labor shortage and will continue to do so going into the future. One of many driving factors behind this automation growth is that the convergence of multiple technologies — including AI, robotics, and machine vision — has enabled the industry to democratize automation by making it easier to deploy and operate.
Automated Inspection Starts with CAD
One significant way that the barrier has been lowered when it comes to automation adoption is the rise in robotic off-the-shelf solutions designed for specific applications or tasks that incorporate several different technologies. “Blind” robots, for instance, can still accomplish many pre-programmed and repeatable tasks, but adding machine vision adds substantial flexibility while opening the door to new applications. Bin picking, for example, combines machine vision — typically a 3D camera — a robotic manipulator, and software to automate material handling tasks. AI-based algorithms can also be incorporated into the software, which allows the system to recognize and classify mixed and new object types, making it a more flexible system.
Other systems on the market today take it even further by leveraging not only 2D and 3D machine vision technologies and robotics, but also AI-enabled software and intelligent robotic planning capabilities that simplify the deployment of a new inspection application, even when dealing with complex parts. End users can define inspection requirements, simply and intuitively, directly on a CAD model of a part in their own software. The system then automatically creates an inspection plan by factoring in part geometry, material properties, camera illumination angles, optimum trajectories, and collision avoidance. If a CAD model of the part does not exist, the system can capture a 3D scan of the part that can be used to define the inspection (Figure 1).
The software also automatically extracts for each inspection requirement specific geometric, PMI (product manufacturing information), and other semantic information defined on the CAD model; this information is then passed to corresponding pre-trained neural networks used to perform the inspection tasks, which provides context for the proper inspection scheme and simplifies the whole process. The software then dictates where a camera with multiple lighting elements mounted on a robotic arm should move to optimally capture images of the part from multiple angles. These images, together with the acquisition parameters and the part geometry are used by the AI software to detect and classify potential defects (Figure 2).
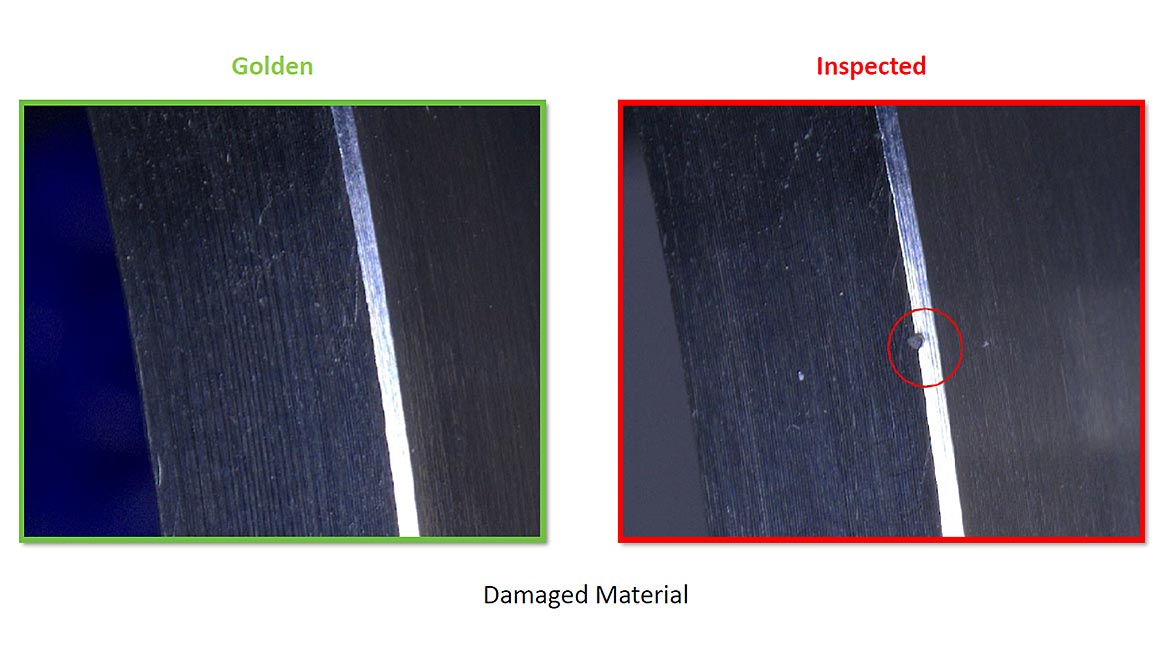
Figure 2: Deep learning-based inspection systems can identify defects such as rust or damaged materials on metal parts.
Empowering AI software to dictate image acquisition and lighting parameters marks a significant leap beyond other AI inspection solutions that merely process the images handed to them. By mastering and adjusting the ideal viewing and lighting angles for an image, it becomes possible to decisively influence whether critical defect information is captured or missed.
Conquering Aerospace Disc Inspection Challenges
Ease-of-use represents an increasingly important priority in automation today, and a hybrid, CAD-based inspection system that automatically plans and maneuvers a camera to capture optimal images of parts allows end users to set up inspection applications quickly and easily. For example, a leading supplier of aerospace technologies recently turned to automation for the inspection of metal discs for jet engine turbines, which must have zero defects upon delivery. Previously, the company relied on human workers to inspect the parts. It took an employee approximately one hour to fully inspect the disc for even the smallest flaws — and the company felt that human inspection was the only means to produce fully defect-free parts since most automated solutions cannot independently verify products and assemblies for every defect.
Human inspection of the expensive, shiny discs eventually proved difficult, however, as the mirrored, reflective metal made it difficult for inspectors to see defects. The higher number of failure points, complex geometries, and product variety made it hard for employees to find every flaw; the work was also monotonous, and, over an eight-hour shift, accuracy began to drop and the defects that made it through the inspection could potentially impact the efficiency of a turbine.
As a result, the company decided to research automated inspection systems capable of doing the job. Many solutions were reviewed, but most could not detect all defects on parts with complex geometries or were extremely sensitive to reflection, negatively impacting overall results. Using a system based on fixed 2D cameras would not be feasible either, since obtaining accurate views in all dimensions would require approximately 600 cameras for each part to cover their geometry complexity. Instead, the company deployed a hybrid system leveraging classic machine vision, deep learning, robotics, and intelligent inspection planning to address all concerns.
After deploying the first system, the company cut disc inspection time from one hour to approximately 12 minutes. Because the system uses deep learning algorithms to differentiate between different types of defects such as scratches or oil smudges, the system dramatically eliminated the identification of false defects while constantly improving as more parts are being inspected. In addition, the system captures all defects and inspection results, performs root-cause analysis, and applies analytics to research failures, which allowed the company to implement a continuous improvement process driven by AI algorithms.
AI: A Powerful Tool for the Toolbox
No single system or technology will ever fit all applications, of course, as each application is unique and requires a detailed analysis and competent system design to achieve any level of success. It’s important to remember this when it comes to research or specifying a technology or system for a given task — and this includes AI.
As individual technologies continue to advance, overall automation system capabilities will continue to expand. For example, open-platform solutions that leverage AI, machine vision, and robotics to optimize image acquisition and lighting parameters for a given part can adapt to varied inspection needs across many industries. Systems such as these make automation easier to deploy across disparate applications, helping to drive increases in productivity, throughput, and revenue.