What started as a simple home repair project ended with multiple trips to the hardware store, cursing in the aisles, and a vow to never buy from a specific manufacturer ever again.
The culprit? A single defective bolt, which had evaded quality inspection and been packaged, shipped, and unfortunately purchased by me. The product packaging, installation instructions, and final functionality were all exemplary. But a single defective bolt, which costs only pennies in the product’s bill of materials, was enough to sour me on the whole experience.
Manufacturers and brand owners are under tremendous pressure to ensure premium end-to-end product quality, especially as consumers increasingly demand perfection. And a great deal of that product quality pressure still falls on human visual inspection.
Humans vs. Machines
Every few days, there’s a new headline warning readers that artificial intelligence (AI) is poised to take jobs from humans. Undoubtedly, there are tasks where machines are more cost-effective and efficient than humans. Buttons replaced the elevator operator, and machine vision-powered robotics has significantly changed large scale manufacturing by taking on many dull, dirty, and dangerous tasks.

End-to-end quality means that even a defect in the smallest component, including a bolt that costs pennies, can significantly influence consumer brand impression. Image Source: Pleora (Click on the image to enlarge.)
For quality inspection, it’s not quite that simple. Machine vision brings speed, accuracy, and repeatability that we simply can’t match as humans. However, many of the advances in automation technologies, including traditional computer vision and more advanced machine learning and deep learning, really aim to replicate what we do very well as humans.
We excel at sensing differences through sight, touch, and even smell depending on the product. We can distinguish subtle flaws, variations, or differences and can adjust when faced with unpredictability. Humans are also easily trained. We learn by example and can adapt to new products or requirements. Unfortunately, unlike machines, we get tired, bored, distracted, and can make subjective decisions. As a result, most manual inspection tasks typically exhibit error rates of 20-30 percent.
Considering these error rates, and the resulting waste, safety risks, and costs, there’s an obvious opportunity to leverage technology advances to aid human inspectors and their decision making.
AI-Powered Decision Support
Adding automated decision-support for human operators helps speed visual inspection rates and improve end-to-end product quality. It also provides more qualitative product evaluation to ensure manufacturing processes are repeatable, traceable and can be consistently replicated across multiple facilities. As consumers increasingly demand more customized products, human inspection can be more cost-effective and adaptive than automated processes for on-demand and low volume manufacturing.
New visual inspection systems leveraging advances in no-code algorithm development and edge processing platforms make it easier and more cost-effective for manufacturers to add AI decision-support for in-progress and finished goods. These systems provide pre-packaged inspection skills that can be easily customized and retrained for specific requirements, including verifying components, inspecting labelling, checking assembly, and digitizing work instructions.
As a fully integrated tabletop system, the operator can use the camera, edge processing, and display to acquire images and create an AI model. Users don’t need to switch between cameras, PCs or cloud-based systems; making the system easy-to-use, highly cost-effective, and all in a smaller footprint.
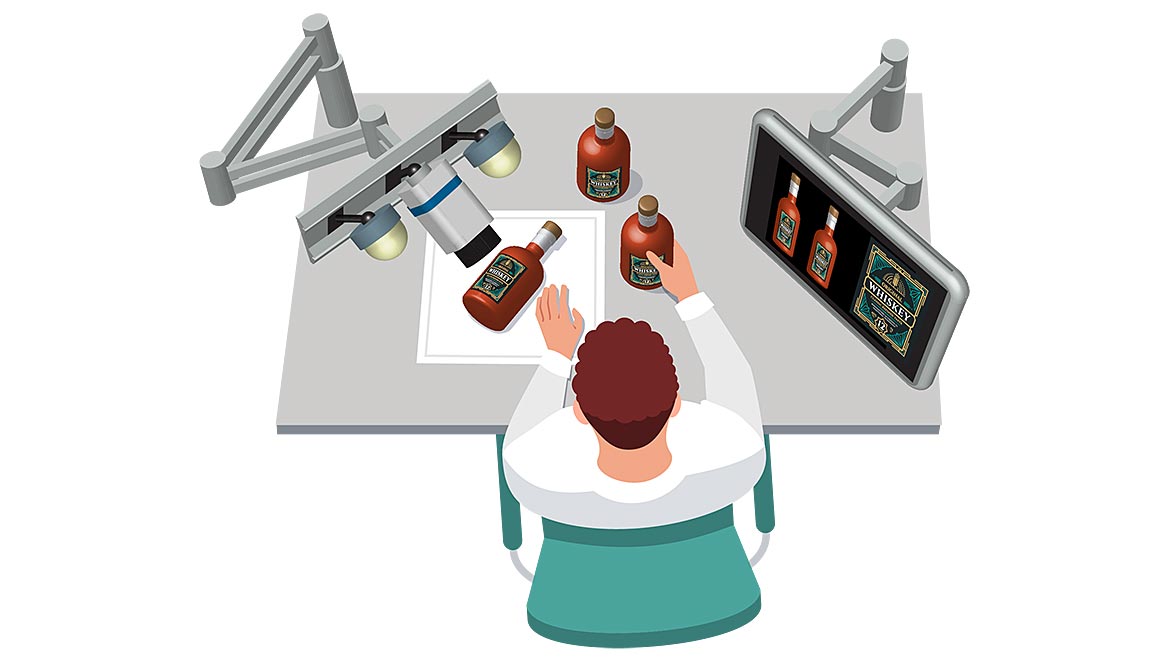
A fully integrated visual inspection system includes a camera, display, edge processing, and pre-packaged common inspection skills, while no code development platforms enable easy customization for unique requirements. Image Source: Pleora (Click on the image to enlarge.)
No Code Advantages
One of the most significant hurdles for manufacturers considering implementing an AI-based inspection system is algorithm development. There is a perception among many that AI development, training, and deployment for manufacturing applications requires specialized skills or expensive consulting.
New no code platforms aim to empower the end-user – including quality managers, operators, and inspectors – to design their own automation workflows. No code block-based platforms allow any end-user to easily design AI and computer models to automate tasks, such as visual inspection, digital work instructions, automated counting and measurement. Pre-packaged inspection skills for common visual inspection requirements can be quickly trained and deployed, without requiring programming language expertise. With just a few image examples, an end-user can build an AI model that distinguishes between ‘good’ and ‘bad.’ More experienced developers can use the same software platform to customize pre-packaged inspection skills, or leverage open source machine vision and AI code as a starting point to build quality checks and workflows for unique requirements.
Augmented Reality And Image Comparison
One of the fastest ways manufacturers can add AI decision-support into processes is with camera-based augmented reality. Augmented reality provides a real-time view of a product on a display monitor, with computer generated graphics to help guide an operator. The system provides immediate visual feedback to the user to verify results during assembly and inspection steps.
In one example, a distillery is using augmented reality in its labelling process. The company operates in a highly competitive market, and its unique bottle and labelling is a key differentiator for consumers as they scan a crowded store shelf. Just like my bolt example, the label represents an incredibly small portion of the manufacturing costs, but if it’s misaligned a consumer may question product quality and move to the next item on the shelf.
In this application, an automated system places the main label on the bottle. It’s then up to a human operator to accurately place a smaller, transparent medallion label onto the bottle that aligns with the corresponding visual brand elements on the main label and cap. With a few different products, fast-changing production schedules, and varying packaging requirements, it’s uneconomical to consider automating this secondary manual labelling process.
The visual inspection system stores a “golden master” image for what each product should look like when it reaches the store shelf. The operator places the bottle under the camera-based system, and a “golden master” image overlay guides the user’s placement of the secondary label in real-time. The system is tuned with thresholds to accept a margin of error, but to the human eye the labels all appear perfectly aligned.
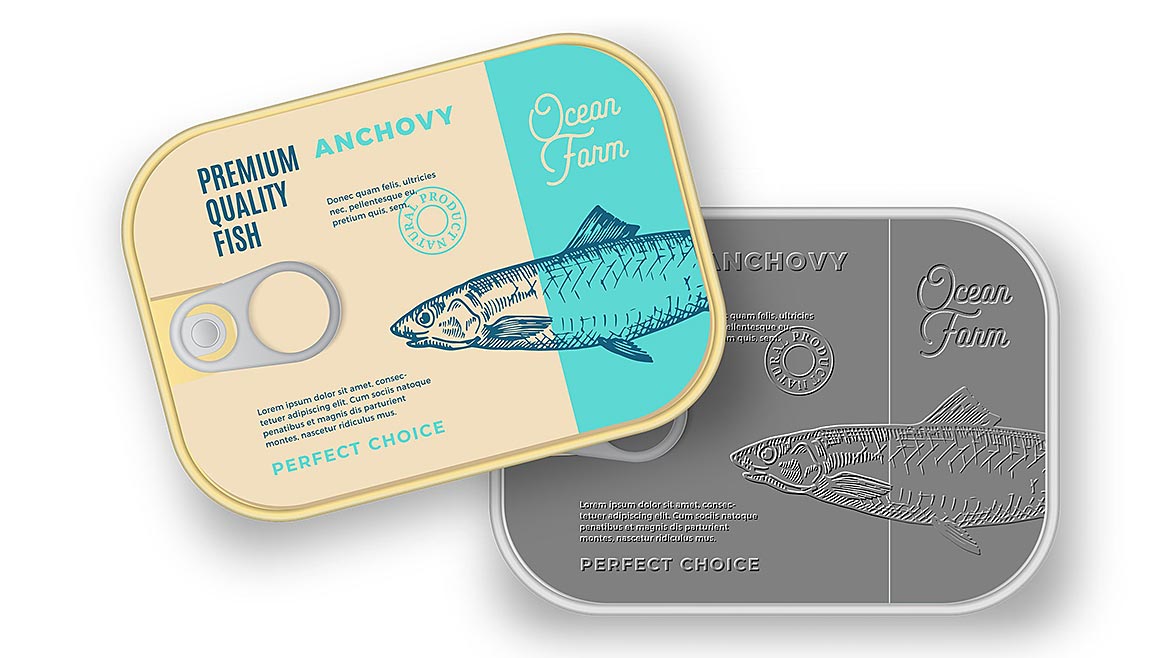
Augmented reality and image compare skills help guide and operator during work-in-process and final assembly and inspection steps. Image Source: Pleora (Click on the image to enlarge.)
The systems adds a few seconds to the overall manufacturing process, but saves the company time and money if labels have to be removed and realigned. Augmented reality also helps speed training for new operators, and removes human subjectivity from the process. If the manufacturer changes the bottle or labelling, with the “no code” platform an operator or quality manager can easily redesign and train their own AI model and deploy it to edge processing integrated in the system.
Similarly, image compare capabilities are used to match the approved layout and final production for electronic assembly applications. The system quickly compares the placement of components on a circuit board, and highlights differences and deviations for the human inspector before it moves to the next step in the manufacturing process or to final packaging. The system is also used by the manufacturer for quality checks on incoming components from suppliers.
In addition, the manufacturer uses the system to capture and save an image of every board. This data is shared with traceability systems for inventory, shipment management, and batch tracking, as well as other business processes including root cause analysis for defects.
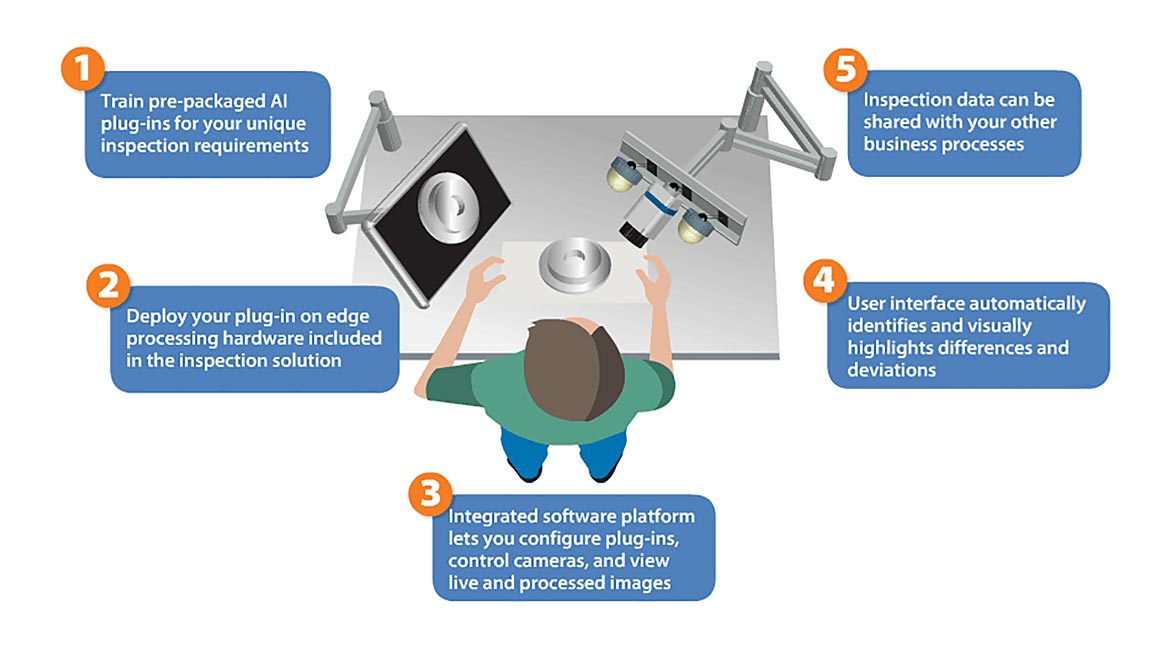
Image Source: Pleora (Click on the image to enlarge.)
Changing Visual Inspection
Adding decision-support and automating processes for visual inspection and assembly helps ensure consistency. The technology can aid a tired operator at the end of a shift, a new employee who is unsure what makes a product ‘good’ or ‘bad,’ and speed inspection and manufacturing processes. It lets manufacturers take advantage of new technologies for processes where automation is cost prohibitive, including low volume production.
As part of a wider Industry 4.0 initiative, automation and decision-support tools for visual inspection can help provide real-time process visibility to drive data-based decisions. Maybe it would have even saved me a few hours of frustration that day at the hardware store.