In the past, machine vision operated on rule-based programming. Traditional rule-based machine vision is powerful, and it’s particularly reliable for inspection applications in controlled environments where defects are somewhat predictable.
But what happens when environments are not so controlled? That’s where edge learning AI technology comes into play.
The role of machine vision in quality assurance is simple: it helps machines perform tasks and identify the systems around them. Edge learning is a type of artificial intelligence (AI) technology that is making machine vision systems easier to use and more powerful.
The main difference between edge learning and other types of AI is that the processing happens “at the edge,” on the vision system, instead of on another device or an external computer. As a result, the combination of machine vision and edge learning is a powerful tool that can aid the manufacturing process in numerous ways.
The Power of AI Without Complexity
Edge learning can play a pivotal role when machine vision systems are implemented in manufacturing: it rapidly learns and adapts to the needs of the production line, optimizing many inspection and sortation applications.
Thanks to pre-trained algorithms built into the hardware, edge learning systems are extremely easy to set up. Because they need fewer training images to “learn from,” setup is much faster compared to traditional rule-based machine vision. It’s incredibly quick and simple to implement, with no prior machine vision or programming knowledge required.
Compared to traditional rule-based systems, edge learning is fast, accurate, and straightforward. As a result, it helps increase productivity, optimize throughput, enhance product quality, reduce costs, cut back waste, and solve complex tasks in minutes.
For countless manufacturers, edge learning is already making an incredible difference. It helps optimize production lines, positively impacting the manufacturing process at large. In short:
- Incorporating an automatic system to correctly identify defects helps to speed up factory lines, accelerate production processes, and produce more.
- Traditional machine vision requires extensive training and programming, but edge learning can solve complex tasks in minutes.
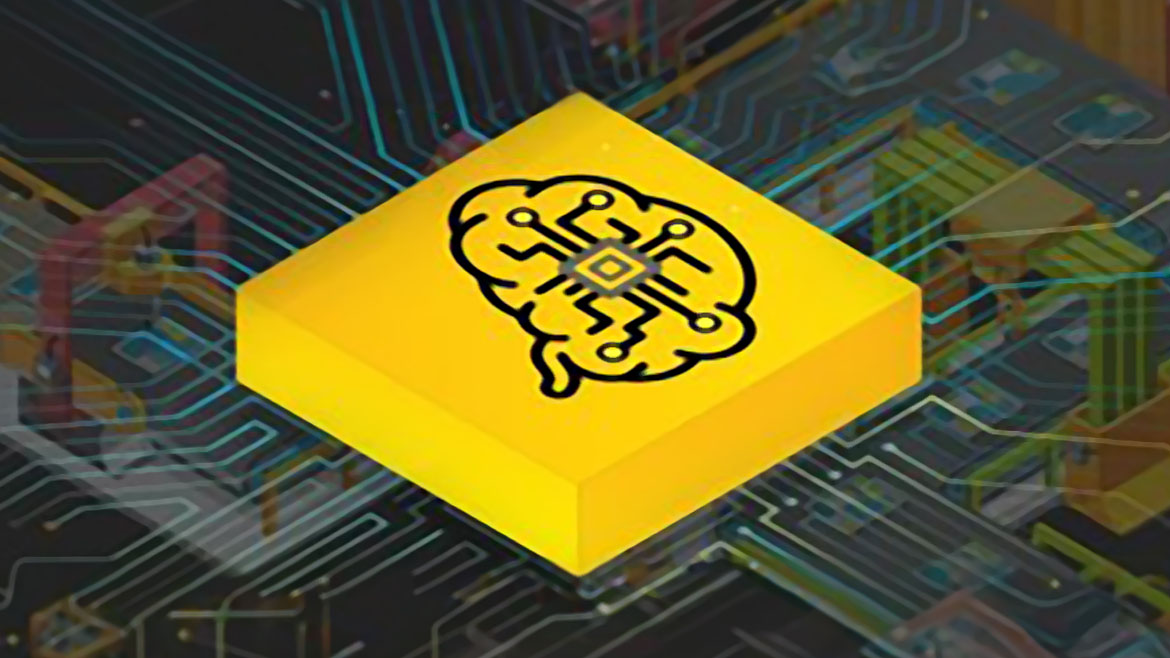
Industries That Benefit from Edge Learning
Edge learning shines in applications where no two defects look the same, since it handles variation better than traditional machine vision technology.
1. Automotive
One application that demonstrates the advantages of edge learning is the inspection of wire connectors in automotive manufacturing. Tightly secured, durable connections are important for long-term reliability, so these connectors need to be carefully inspected before vehicle production moves forward.
However, inspection can be a challenge because these wire connectors have a wide range of clips, snaps, and other ways of joining together. They’re also typically made of black or dark-colored plastic, making it difficult for some machine vision systems to see intricate details.
Rule-based inspection systems can struggle to accurately identify all the possible variations in loose, broken, or missing wire connections, resulting in problems further down the production line.
A system using edge learning, though, can learn to consistently identify defects to prevent them from passing through the production line. Thanks to the built-in AI, it can catch problematic connections without having to be told exactly what types of defects to look for.
2. Medical and Pharmaceuticals
In pharmaceutical and medical device applications, precision is crucial for patient safety. Edge learning helps manufacturers live up to that promise of safety by drastically reducing the risk of flawed products or medicines making it into circulation.
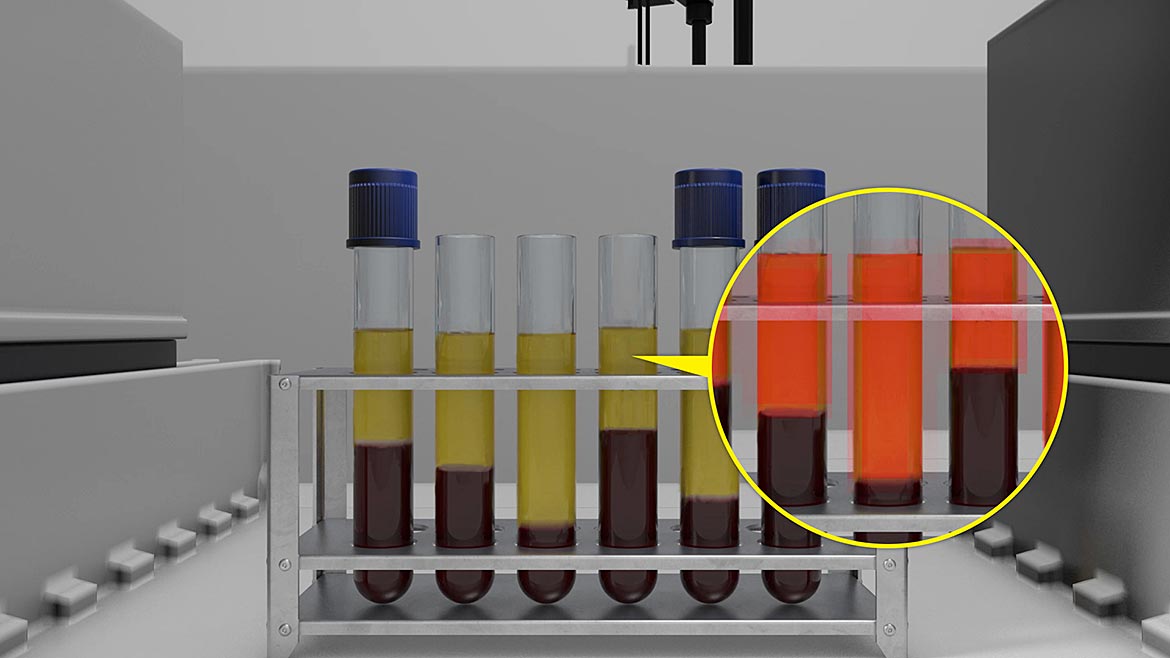
In vaccine production, for example, glass vials need to be consistently filled to a precise level, and machine vision-based inspection is essential in verifying that dosage. Traditional rule-based machine vision can struggle to achieve consistent detection rates due to reflection or glare off the glass and its contents.
Thanks to flexible AI identification algorithms, edge learning correctly detects fill levels while overlooking glare and reflection. The AI successfully detects good vials, accounts for subtle variations, and ignores other challenges such as reflectivity.
3. Food and Beverage Packaging
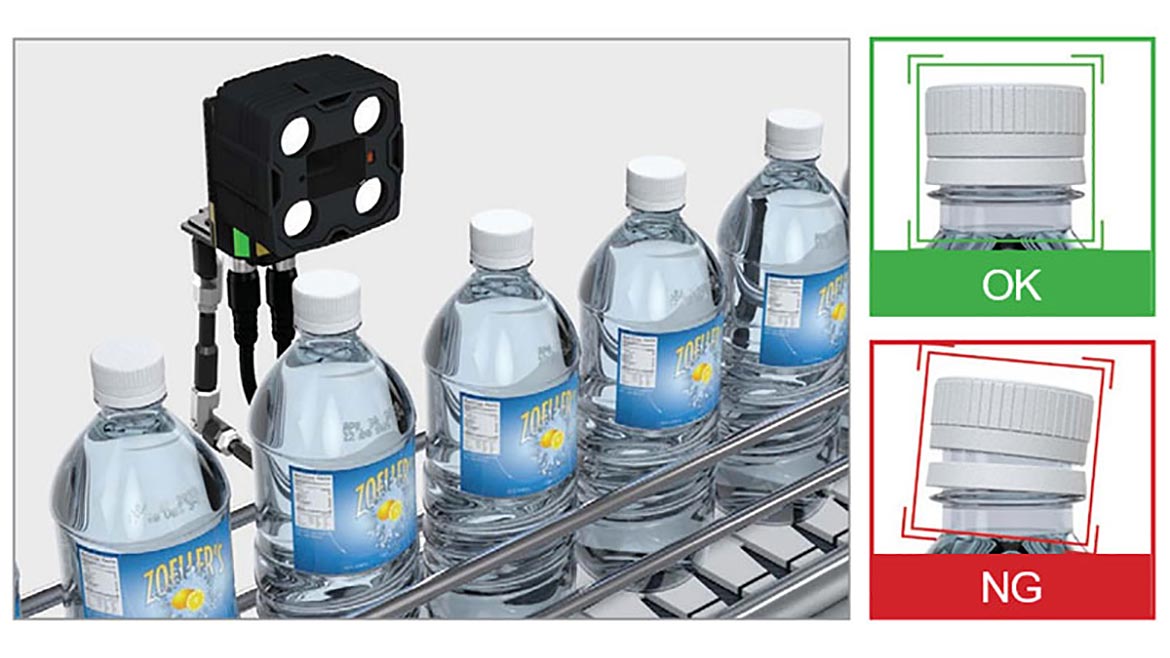
A water bottle production line can offer a wide variety of defects that edge learning is well-suited to addressing.
- Unscrewed or misaligned bottle caps
- Scratches on the bottle
- Tears in the plastic
- Incorrect label information
- Faulty fill levels
Edge learning systems can identify these types of problems quickly and efficiently – and when packaging changes, edge learning can be quickly retrained with a new set of “good” and “bad” training images – no programming expertise needed.
Traditional rule-based systems can be reprogrammed, but only by experienced machine vision engineers.
4. Assembly Verification
Edge learning is now being used in applications where inspection has typically been done manually, like final assembly check applications: tasks that were sometimes considered too difficult to automate.
With edge learning systems, inspection and identification happens more consistently, more reliably, and more quickly than manual inspection or traditional machine vision systems.
Using this AI tool on a final assembly check production line, for example, ensures that the correct components are within a box, container, package, or kit, where the contents may not always be arranged in the same way.
Traditional rule-based technology can sometimes be used in applications like this, but only with time-consuming programming.
Edge learning combines the precision of human inspection with the reliability of artificial intelligence.
Edge Learning Reduces Costs
The advantages of edge learning translate into real cost savings in multiple ways.
- Defective products or parts are a significant expense for any production line. Better inspection tools reduce waste and drastically cut costs.
- Edge learning reduces the time required to switch over a line to a new product or package, providing cost savings by shortening expensive downtime.
- Additional inspection solutions cut costs by identifying defects earlier in the production process, before additional time and materials are used on bad parts.
- Edge learning provides better accuracy than human operators. Most inspectors can only focus for 15-20 minutes, resulting in inconsistencies between production lines.
When switching to edge learning, manufacturers can be surprised to learn how quickly yield and throughput improve.
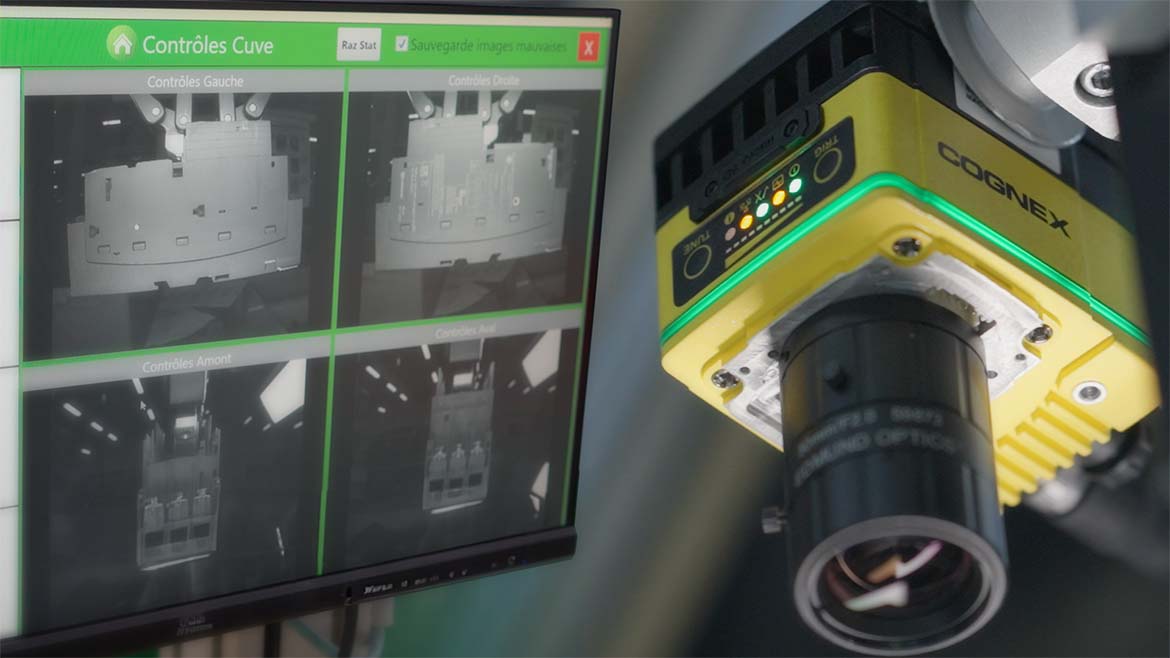
Four Hours to Production: Edge Learning Quickly Makes a Difference at Schneider Electric
Leading electronics manufacturer Schneider Electric specializes in the digital transformation of energy management and automation. They recently discovered that edge learning can play a key role in their ongoing transformation as they invest in smart factories that use connected devices, machinery, and production systems to continuously collect data.
Traditionally, this manufacturer relied on rule-based machine vision. The system worked, but it produced inconsistent quality results, along with a higher than acceptable level of false rejects.
Among other applications, Schneider Electric implemented edge learning to conduct quality inspections and identify presence/absence on pad and spring components for assembly.
According to Laurent Chantoiseau, GSC Europe Data & Analytics Director at Schneider Electric, “Edge learning solutions are incredibly easy to set up; in less than 4 hours for a simple use case, we can have an application ready for production.”
Schneider Electric has a variety of applications that range from simple to complex, and edge learning offers quick and easy solutions to a multitude of complex production challenges. As edge learning is successfully implemented into Schneider Electric’s factories, the company refers to them as “smart factories.” Schneider’s smart factories learn and adapt in real or near real-time, increasing efficiency, improving quality, and lowering costs.
Conclusion
Edge learning’s ability to solve incredibly challenging production problems with simplicity is making a difference in the manufacturing world today.
- The technology performs exceptionally with minimal training, solving challenges in minutes.
- It greatly boosts inspection performance, accurately detecting subtle defects.
- It’s fast and easy to integrate into any manufacturing environment, with no prior machine vision knowledge required.
- The power of edge learning, combined with machine vision, becomes a game changer for OEMs and manufacturers across industries.
Artificial intelligence has come a long way, and it’s now a powerful tool to harness in manufacturing. Thanks to edge learning, even smaller manufacturers who thought AI solutions were out of reach can now start taking advantage.