Vision systems have developed a checkered reputation as integrating hardware and getting consistent results remain a challenge, but robust solutions take operations to the next level.
Manufacturers have been working towards built-in quality for years, developing roadmaps for the path to zero defects largely focused on quality systems compliance, process failure mode reviews, and incremental inspections. Vision systems long ago emerged as the go-to for automated quality inspection, but walk around any production facility, and you’re certain to find at least one of these systems in bypass due to a high incidence of false negatives / false positives, general frustration with stability, lack of in-house vision expertise or a combination of these reasons.
Meanwhile, labor shortages continue to put pressure on manufacturers, so it’s remarkable how many facilities still have as much as 20% of their workforce dedicated to manual inspection. Imagine the value to be unlocked by embracing a plan for Quality 4.0 with automated in-line inspections combined with AI process analytics that enable true process optimization. Now that’s built-in quality.
With the myriad of machine vision solutions available and a clear path to ROI due to cost of quality and labor shortages, it is not surprising that a recent LNS Research report indicated that 43% of respondents either had vision systems deployed or are planning to do so in the next one to three years. (Reference: https://blog.lnsresearch.com/quality-4.0-in-operation-2030-the-journey-to-zero)
If we can bridge the confidence gap between underperforming legacy vision systems and manufacturers’ needs today, the rate of adoption is sure to grow exponentially. This article will explore five key considerations for selecting an integrated machine vision solution.
- Understanding critical quality factors for inspection
- Selecting the correct hardware and software for the product and process
- Ensuring solution stability with changing conditions on the machine or line
- Assessing system performance vs. needs
- Closing the loop for built-in quality
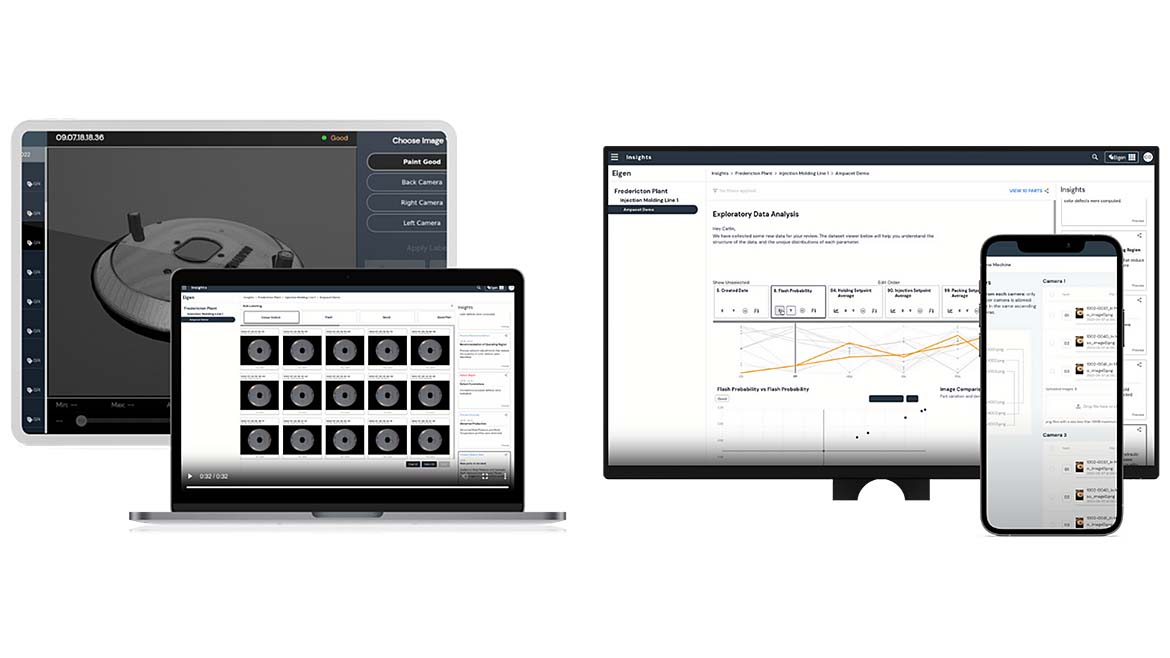
Understanding Critical Quality Factors for Inspection
To achieve the intended value from a machine vision deployment, it is important to first identify what is truly critical to quality in a manufacturing process. We have conducted many process reviews where a manufacturer expects a single vision solution to be able to capture product or part dimensions, assembly features, and appearance defects on complex shapes without changing the way parts are handled. While these qualifiers represent the ideal state, they often require different sensors or lighting strategies depending on the features to be verified. Some may even require additional automation to articulate parts for full coverage or to make defects visible. The result can be a project with excessive complexity or cost to get off the ground.
What if we take a step back and assess what is truly critical to quality? What are the key features or attributes that must be verified to ensure a good product leaves the factory? Do we understand the minimum size or defect criteria required to be inspected? It is amazing how many times this is not fully understood, which inevitably raises the question - how do we evaluate the success of the vision system?
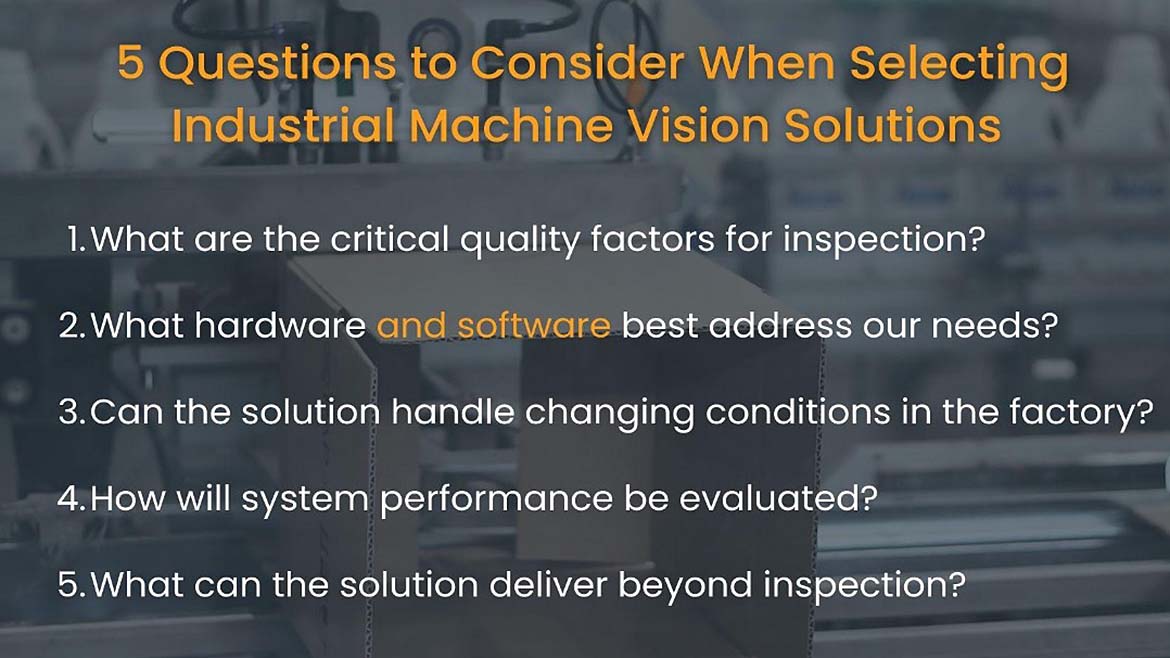
Selecting the Correct Hardware & Software for the Product and Process
For most vision applications, hardware selection entails much more than a camera. It is typically an integrated system of camera(s), lighting, an edge computer and HMI designed for the specific product and process. End-to-end vision software packages support system configuration and integration, the visualization of image data, and the development of algorithms or training of AI models depending on the use case. It may seem easiest to select a camera brand and use its software, but camera manufacturer software often lacks functionality and integrating with other data sources is not readily available or possible with some locked platforms.
An entire article could be written on camera selection alone. The most common are 2D area-scan and line-scan cameras, but can also include 3D profilers where height data is critical, or multispectral sensors for applications that go beyond the visible spectrum. Machine geometry largely limits the locations available for camera mounting, driving many applications to require multiple cameras to get full coverage. Regardless of sensor type, it is critical to select field of view, pixel resolution, and imaging rates aligned to the defect sizes and line speed of the process.
Lighting remains one of the most challenging areas in vision deployments. As with cameras, the lighting type must be aligned to the specific defect types to be identified. One of the more common errors driving inconsistencies is the use of bright field lighting on surfaces that reflect too much light into the camera. A simple change to dark-field illumination often improves the image quality and defect visibility dramatically. In other cases, a series of structured lights with multiple image captures may even be required.
As vision applications increase in complexity, multiple types of cameras and lighting can often be combined for a more comprehensive inspection. This could be a combination of infrared and 2D line-scan on a plastic film line, or perhaps a 3D profiler with 2D area-scan to evaluate a glue bead or the surface of a stamped part. In these cases, selecting GigE Vision cameras ensures compatibility with software packages with more advanced capabilities than many camera manufacturers provide. This further unlocks the ability to start to consolidate and trend data across various inspection points in a line or facility.
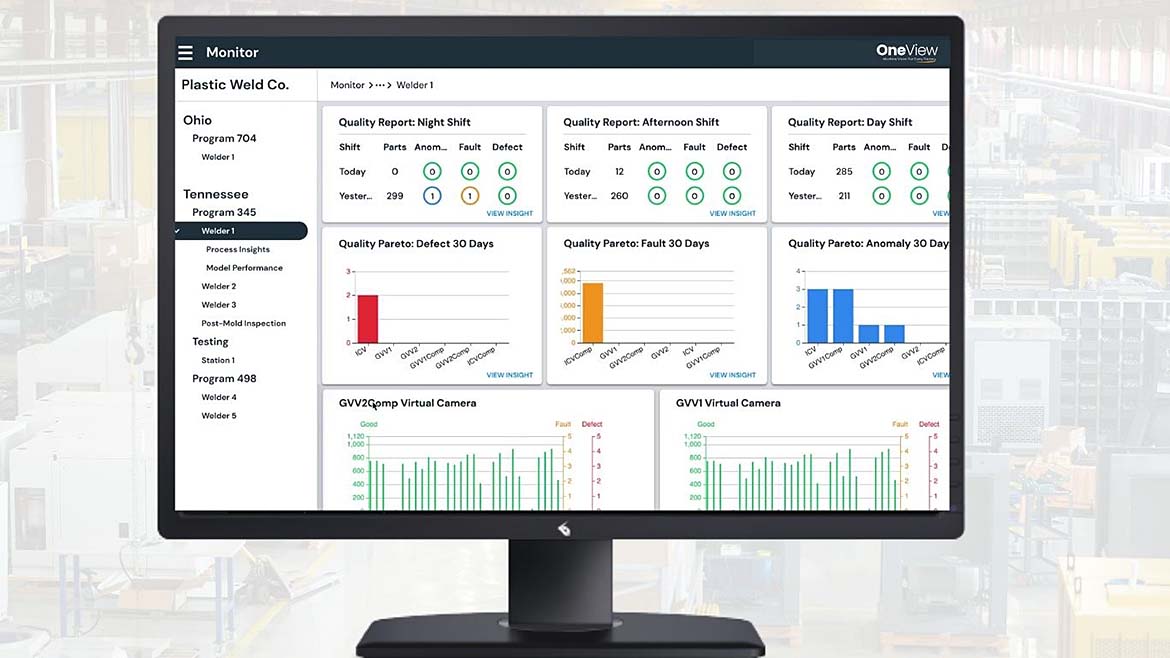
Ensuring Stability With Changing Conditions on the Line
On more complex vision solution installations, it is recommended to complete some offline testing with the camera/lighting configuration and samples across the full range of defects to validate visibility before deployment. Unfortunately, no amount of offline testing can fully mitigate some of the variation introduced when installing in production. Variable ambient lighting, vibrations, image trigger timing, airborne debris, camera bumps, and part position are all common issues that impact the performance of vision systems over time. These are often the reasons why systems get bypassed after weeks or months of dealing with inconsistent performance.
Almost every plant we visit has a history of failed vision projects that the quality and production teams don’t want to repeat. Fortunately, new software tools now exist that help to reduce the impact of process-induced noise or variation. Our vision solutions feature image standardization and compare-to-CAD processes that run in real-time at the machine or process on the shop floor. Cameras that are bumped during production or a maintenance event can be brought back into position via a live stream on a nearby HMI. Advances in AI/ML models also help to counteract some of these effects, while understanding the variation across images to dial in thresholds for system performance and stability. When considering new vision solutions, while selecting hardware is important, it’s equally critical to understand the software capabilities that support system stability, ease-of-use, integration with other systems and expansion over time.
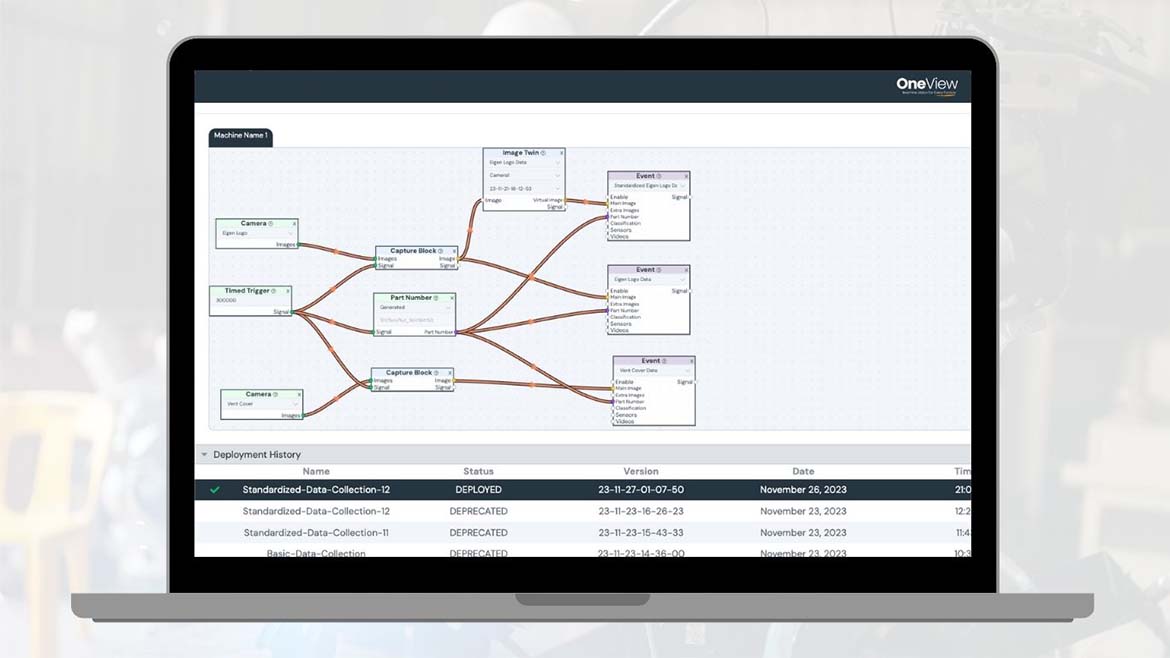
Assessing Vision System Performance vs. Needs
Traditional rules-based vision algorithms can typically be evaluated with a known set of good and bad products to verify compliance. The approach with deep learning solutions is quite different. It typically starts with a large image collection to understand the full range of defects and the frequency at which they occur. Initial models can then be trained and deployed in parallel to the existing inspection to validate classification and provide clarity on parts that may need to be relabeled. This iterative process takes place over a few weeks where previously unobserved elements of product and process variation are often revealed in the data set. Evaluations of model accuracy typically take the form of a confusion matrix, with incremental reductions in the number of false positives and false negatives over time.
This can be challenging for a quality team accustomed to statistical process control, as refining detection with deep learning is much more iterative with robust models often performing in the 85-90% accuracy range. As deep learning is often used for the more variable subjective defect classes (ie. aesthetic surface defects), accuracy in this range may still well exceed the capabilities of a manual visual inspection (75-80% best case). Deployments with AI often get stuck being evaluated against traditional quality statistics, when they should be assessed in terms of meeting the intended needs. Labor can then be reassigned to higher-value tasks and processes. If well maintained, performance will only continue to improve with time.
Closing the Loop for Built-in Quality
Vision systems have been traditionally deployed in isolated processes, providing an output at the specific station but without more broad integration. This is a major gap and an opportunity to drive quality improvement. When vision data is combined with process analytics at the edge, we can start to leverage AI to not only detect defects but start to find correlations between the image and process parameters influencing quality. When more vision systems on the line can be centrally managed in a single platform with this process data, it unlocks the potential to detect trends earlier, allowing teams to prevent defects that may have been generated upstream. Planning for this upfront by selecting vision hardware and software that support advanced process integration will open the possibilities that drive Quality 4.0.